Understanding the Role of Recipe Datasets in Data Analysis
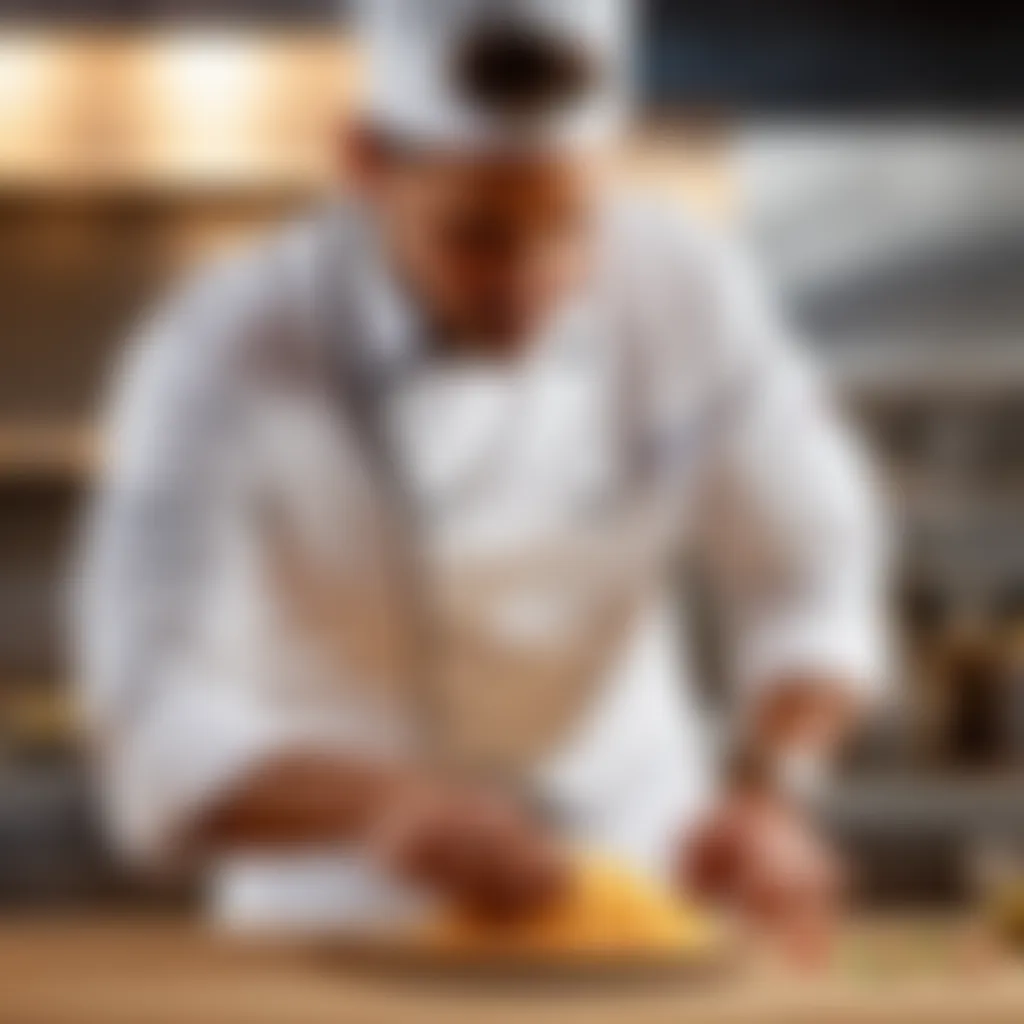
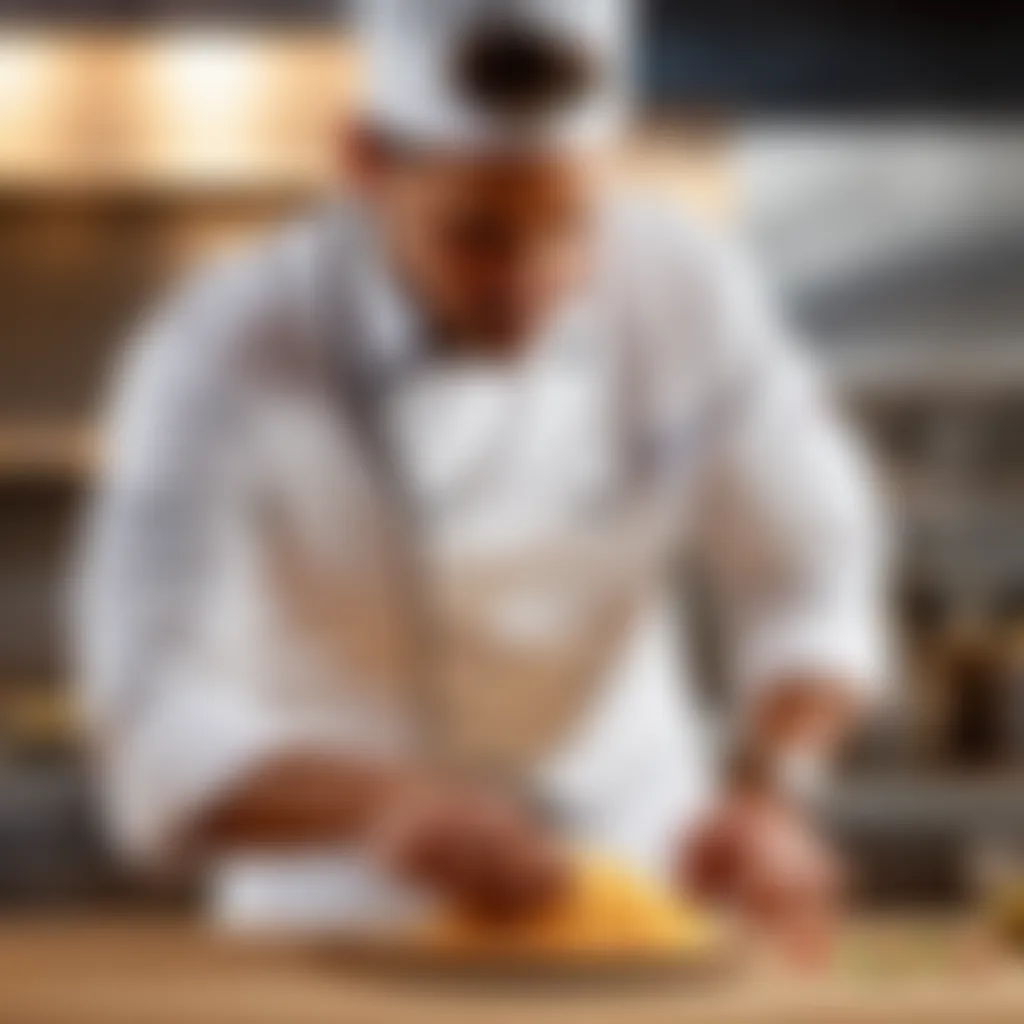
Intro
In today's world, where culinary traditions meet cutting-edge technology, recipe datasets stand as unsung heroes in data analysis. These datasets are treasure troves of information, revealing not just the ingredients and methods but also the cultural underpinnings of various cuisines. By dissecting these datasets, we unlock insights that go far beyond the kitchen, impacting food industries, nutrition guidelines, and even consumer behavior.
This article serves as a thorough exploration of the widely underestimated importance of recipe datasets. From understanding culinary trends to navigating the complexities of flavors and textures, these datasets transform the way we perceive recipes. With a systematic approach, we will cover essential elements such as ingredients, preparation methods, technical aspects, cooking processes, and navigation of common challenges. Thus, we will guide budding data analysts and culinary enthusiasts alike to appreciate the vast potential that lies in the convergence of food and data.
Ingredients:
When it comes to understanding cooking through data, the first step is gathering reliable and exact information about ingredients. To illustrate this, let's consider a straightforward yet delicious recipe to make a classic spaghetti aglio e olio. Here’s a detailed list of ingredients with precise measurements:
- Spaghetti: 400 grams
- Garlic: 6 cloves, sliced thinly
- Olive oil: 120 milliliters
- Red pepper flakes: 1 teaspoon, or to taste
- Parsley: 1/4 cup, finely chopped
- Salt: to taste
- Parmesan cheese: freshly grated, for serving (optional)
By maintaining a rigorous and detailed account of these ingredients, one doesn’t just follow a recipe; rather, one engages in a data-driven approach to culinary arts that mirrors the precision of data analysis.
Preparation Steps:
In any culinary endeavor, preparation is half the battle won. Here’s how you can get ready to whip up that spaghetti aglio e olio:
- Gather your ingredients: Ensure that everything is measured and ready to go before you begin cooking. This eliminates confusion and enhances efficiency.
- Chop the garlic: Slice the garlic cloves thinly. Be cautious; the thinner your slices, the better the distribution of garlic flavor.
- Prepare the parsley: Finely chop the parsley, setting it aside for garnishing later.
- Boil water: In a large pot, boil water and add a generous amount of salt. The water should taste slightly salty like the sea. This enhances the flavor of the pasta.
Having each element systematically prepared aids in a seamless cooking experience, reminiscent of a well-structured data analysis.
Technical Aspects:
Understanding the technical aspects of cooking transforms the process into something akin to scientific experimentation. Consider the following:
- Temperature settings: For the olio, use medium heat when cooking the garlic. It should sizzle gently, releasing its aroma without browning.
- Timing specifics: Cook spaghetti according to package instructions—typically 8 to 10 minutes for al dente. Taste a strand to ensure it’s just right.
- Critical techniques: Emulsifying the pasta with oil is essential. Make sure to reserve some pasta cooking water before draining it to create a silky sauce later.
Cooking Process:
The cooking process can be broken down into detailed sequential steps to ensure clarity in how each component merges into the final dish:
- Cook the Spaghetti: Once the water is boiling, add the spaghetti. Stir occasionally until al dente.
- Prepare the Sauce: In a large skillet, heat olive oil over medium heat. Once it’s hot, add the sliced garlic and red pepper flakes. Stir continuously to avoid burning.
- Combine Pasta and Sauce: Drain the spaghetti, keeping a cup of pasta water. Add the hot pasta to the skillet, mixing it well with the garlic oil. If it seems dry, add a little reserved pasta water to achieve the desired sauciness.
- Finish Up: Toss in chopped parsley and salt to taste. Serve immediately, topped with freshly grated Parmesan if desired.
Remember, the cooking time and conditions may vary, so keep an eye out to adjust accordingly. Your intuition in balancing flavors and observing temperature can make all the difference, similar to how one adapts data analysis techniques based on varying datasets.
Troubleshooting Tips:
No cooking journey is without its bumps. Here are some helpful solutions to potential hurdles:
- If the garlic starts to brown before you add the spaghetti, it's too hot. Lower the heat immediately to prevent bitterness.
- Should your pasta seem too dry, simply add a ladle of reserved pasta water to rehydrate the dish.
- If it needs more flavor, a pinch of salt or a sprinkle of additional red pepper flakes can do wonders.
"Cooking is like data analysis, the more you know the basic principles, the more creative you can be in your approach."
As we continue to navigate the intricate terrain that binds culinary arts with data analysis, our understanding only deepens. Recipe datasets illuminate patterns and practices that aren’t immediately visible, allowing us to not just create, but also to learn.
Understanding Recipe Datasets
Understanding recipe datasets is crucial for grasping the broader implications of culinary data analysis. These datasets not only encapsulate recipes but also convey cultural significance, flavor profiles, and nutritional values embedded within the food industry. They act like windows into the culinary world, revealing trends and consumer preferences that might otherwise go unnoticed. The analysis of these datasets can facilitate targeted product development, menu optimization, and even nutritional research, enriching both culinary practice and data science.
Defining Recipe Datasets
Recipe datasets consist of collections of recipes, which can include ingredients, preparation methods, cooking times, and nutritional information. It’s worth noting that these datasets can vary significantly in complexity and format. For instance, simple datasets might list ingredients and instructions, while more comprehensive ones could include user reviews, nutritional breakdowns, and even photos of the finished dishes. The fundamental characteristic of a recipe dataset is its structured composition that allows for data analysis and interpretation.
Types of Recipe Datasets
Diving deeper, recipe datasets typically fall into three main categories: structured, unstructured, and hybrid datasets. Each brings its own unique flair to the table in terms of data analysis.
Structured Datasets
Structured datasets are like well-organized cookbooks; they have a clear format that allows easy extraction and analysis of information. They often consist of rows and columns, making them popular for data-driven applications. In this context, the key feature of structured datasets is their predictability. They enable simpler data manipulation and analytics through tools like SQL or data frames in programming languages such as Python.
For instance, a structured dataset might include columns for recipe names, ingredient lists, quantities, and cooking times. This format allows for immediate statistical analysis, which can highlight trends in ingredients or cooking methods. However, one downside is their limited capacity to capture nuanced information such as user interactions and comments.
Unstructured Datasets
Unstructured datasets, on the other hand, resemble a chef's messy workspace scattered with bits and bobs of food preparation. They contain qualitative data, such as user reviews and images, which do not fit neatly into rows and columns. The richness of unstructured datasets lies in their diversity. For a culinary analyst, they provide context and flavor—literally and figuratively—around recipes that structured datasets might miss.
For example, an unstructured dataset may include a collection of social media posts featuring photos of dishes or comments on recipes. Although extracting actionable insights from unstructured data can be complex and time-consuming, it also opens the door to rich, narrative-driven analyses that can enhance understanding of current culinary trends.
Hybrid Datasets
Hybrid datasets bring the best of both worlds, incorporating elements of structured and unstructured data. They often consist of organized recipe components alongside descriptive or qualitative elements, such as user ratings or preparation stories. The flexibility of hybrid datasets is their main advantage, allowing a more holistic view of culinary data.
These datasets can be valuable for creating well-rounded user profiles. For instance, a hybrid dataset might include structured information like ingredient proportions together with user-submitted tips about modifying a recipe. However, managing such datasets may require sophisticated data management techniques, making analysis a bit tricky, yet rewarding.
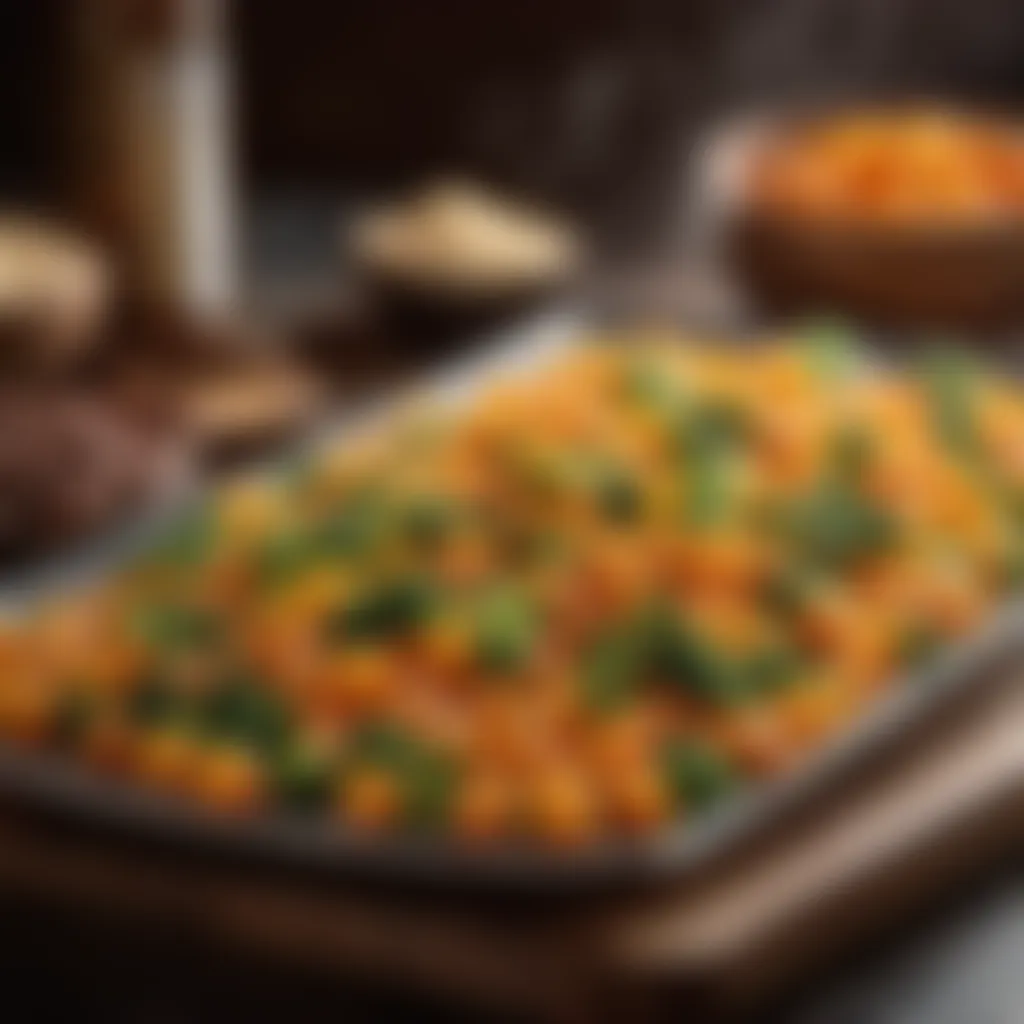
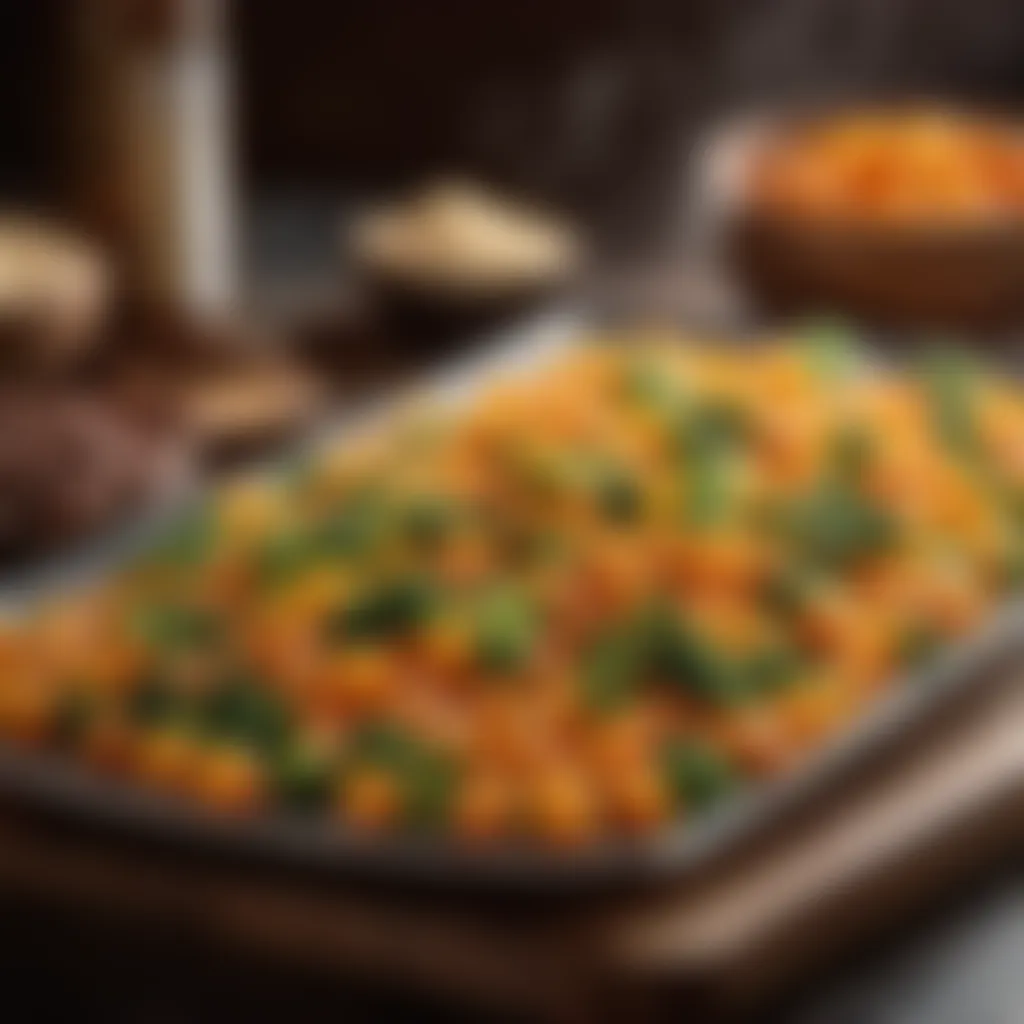
"Recipe datasets, whether structured, unstructured, or hybrid, provide unique paths to understanding food preferences and trends, enriching both culinary practice and consumers' experiences."
By recognizing the fundamental types of recipe datasets, one can better appreciate how they reflect cultural practices, adapt to seasonal trends, and respond to changes in consumer preferences. This foundational understanding is essential for further exploring the intersection of data and culinary arts.
The Role of Data Analysis in Culinary Studies
In the expansive culinary world, there’s more than just a good recipe behind every delightful dish. Data analysis plays a pivotal role in deciphering the intricate relationship between food, culture, and consumer behavior. It’s not merely about numbers and figures; it is about finding patterns and unraveling stories hidden within recipe datasets. The significance of data analysis in culinary studies can’t be overstated, as it allows chefs, food scientists, and culinary experts to glean insights that can enhance culinary practices and cater to evolving consumer preferences.
Interdisciplinary Approaches
Data analysis in culinary studies doesn’t exist in a vacuum. It thrives on interdisciplinary collaboration, combining fields such as nutrition science, sociology, and economics. Each of these domains offers valuable perspectives that can drive more informed cooking decisions and a deeper understanding of food.
- Nutrition Science: Using recipe datasets, nutritionists can analyze the nutritional content of various recipes. This aids in creating healthier meal options that cater to dietary preferences and restrictions. For instance, by examining trends in ingredient choices, they can propose modifications that retain flavor while enhancing health benefits.
- Sociology: Recipe datasets can reveal cultural patterns and traditions surrounding food. Analyzing these datasets helps to understand how different demographics respond to culinary innovations or changes in food habits, such as the rise in vegetarianism or gluten-free diets.
- Economics: By examining the economic implications of recipe ingredients and market trends, culinary analysts can provide insights into cost-effective cooking. This becomes particularly valuable in today's climate, where budgeting is key for many families.
Data analysis, therefore, serves as a bridge across these fields, allowing for comprehensive insights that benefit culinary studies. In short, this interdisciplinary approach enriches culinary practices by ensuring that they are not just tasty but also nutritious and culturally relevant.
Culinary Preferences and Trends
Tastes do change, and understanding these shifts is crucial. Recipe datasets become a treasure trove for analyzing culinary preferences and trends. Whether it’s the sharp rise in plant-based eating or the sudden popularity of ethnic cuisines, data analysis helps to recognize these changes early on.
- Identifying Trends: By tracking which ingredients are increasingly appearing in recipes, data analysts can identify trending flavors or cooking methods. For example, if quinoa starts popping up in more recipes, it signals a growing interest in health-conscious eating.
- Consumer Preferences: Data analysis can also reveal what diners are craving based on past consumption data. When restaurants utilize this information, they can better tailor their menus to meet customer desires, ultimately enhancing the dining experience.
- Influence of Social Media: In today’s tech-savvy world, culinary trends are often sparked by social media influencers. Analysis of social media data alongside recipe datasets gives a clear picture of how certain dishes explode in popularity thanks to viral recipes.
Understanding culinary preferences through data analysis not only helps in meal preparation but also paves the way for innovative dining experiences, addressing the desires of consumers and enriching their relationship with food.
In summary, the interplay between data analysis and culinary studies yields remarkable insights that benefit both the culinary community and consumers. As patterns emerge and preferences evolve, embracing these changes through data-driven decisions can profoundly transform our kitchens and dining tables.
Data Collection Methods for Recipe Datasets
When it comes to tapping into the rich world of recipe datasets, understanding the various methods of data collection is crucial. These methods not only give life to the datasets but also influence the quality and relevance of the information gleaned from them. Effective data collection enables better analysis, which ultimately informs culinary decisions, trends, and innovations.
Effective data collection methods ensure that the information used to analyze culinary patterns is accurate and useful. In today’s data-driven culinary landscape, proper methodologies propel the discovery of new trends and preferences. Therefore, delving into how data is collected can illuminate the underlying factors that shape culinary practices and consumer behavior.
Web Scraping Techniques
One of the most modern approaches to gather recipes is through web scraping. This technique involves extracting data from websites, which is particularly handy for chefs, food bloggers, and culinary researchers alike. By leveraging various tools and programming languages, like Python, one can automatically collect vast amounts of recipe data from numerous culinary websites.
However, as we dive deeper, the conversation about web scraping raises important questions about data quality and ethical considerations. Scanning means ensuring that the extracted data holds value; no one wants a pile of half-baked data, right? Thus, it is essential to implement strategies that filter out unreliable content, such as user-submitted recipes without verification.
- Tools and Languages: Tools like Beautiful Soup or Scrapy are commonly used in Python for scraping tasks.
- Considerations: Before scraping any site, it’s a good practice to check its terms of service. Some sites might restrict automated data collection.
In essence, using web scraping can yield a treasure trove of culinary information, provided one does so responsibly and ethically.
Crowdsourcing Information
On another front, crowdsourcing has become a game-changer in the recipe data collection arena. This method harnesses collective expertise from the masses. Popular platforms such as Reddit and Facebook groups foster a communal space for sharing recipes, tips, and unique cooking methods.
Crowdsourced data offers unparalleled diversity—a melange of kitchen experiences and cultural practices that no single individual could replicate. Moreover, the collaborative nature of crowdsourcing leads to a dynamic flow of information, constantly evolving as users contribute new ideas or refine old ones.
However, it comes with its own sets of challenges. The authenticity of recipes must be scrutinized; just because a recipe is shared doesn’t mean it’s foolproof. To ensure high standards, moderation and guidelines are crucial.
- Benefits of Crowdsourcing:
- Diversity: A wealth of recipes from varied cultural backgrounds.
- Real-time Updates: Fast adaptability to trends and preferences.
Incorporating both web scraping and crowdsourcing into the recipe dataset framework augments the quality and depth of data, leading to insightful analysis and a better understanding of culinary landscapes. It's about gathering data the smart way and ensuring it serves the purpose you intend for it.
Analyzing Recipe Ingredients
Analyzing recipe ingredients is not just about rattling off what goes into a dish; it provides a doorway into understanding the culinary landscape, nutritional value, and even cultural significance of foods. When one digs into ingredients, they unveil a myriad of insights that can influence everything from meal planning to product development. Such an analysis proves invaluable, particularly for those who want to elevate their cooking while being mindful of their health and nutrition.
Nutritional Analysis
Nutritional analysis transforms mere ingredients into health-conscious decisions. For the average housewife, knowing the nutritional content of family meals can significantly impact dietary choices. This analysis accounts for calories, vitamins, minerals, proteins, fats, and carbohydrates, all of which play critical roles in health.
By understanding the nutritional breakdown of common ingredients, one can make informed substitutes. For example, swapping out regular pasta for whole grain options can yield higher fiber content, contributing positively to digestive health.
Moreover, this kind of scrutiny helps in catering to specific dietary needs, such as gluten-free or low-carb diets. Incorporating high-nutrient foods can also enrich meals, making them wholesome and satisfying. When you start cooking with a clear picture of what's going into each dish, you gain an edge in meal creation, taking control of your family's diet.
- Example: Analysis might show that adding spinach to a pasta dish not only enhances taste but also significantly boosts iron and vitamin A intake.
- Considerations: Keep in mind that some foods lose nutrients through cooking; a careful approach is needed to balance flavor and health.
Ultimately, a thorough nutritional analysis encourages a more mindful kitchen, where meals become not just enjoyable but also health-promoting. It grounds the home cook in the virtues of food-based decisions.
Ingredient Pairing Insights
Understanding how ingredients complement one another can elevate a dish from mundane to divine. Ingredient pairing insights focus on harmonizing flavors, textures, and even colors to produce visually appealing and tasty meals. Think about how lemon zest can brighten a rich cream sauce or how rosemary’s earthy notes enhance roasted potatoes.
The art of pairing isn't only about taste; it also considers the chemical reactions between ingredients. For example:
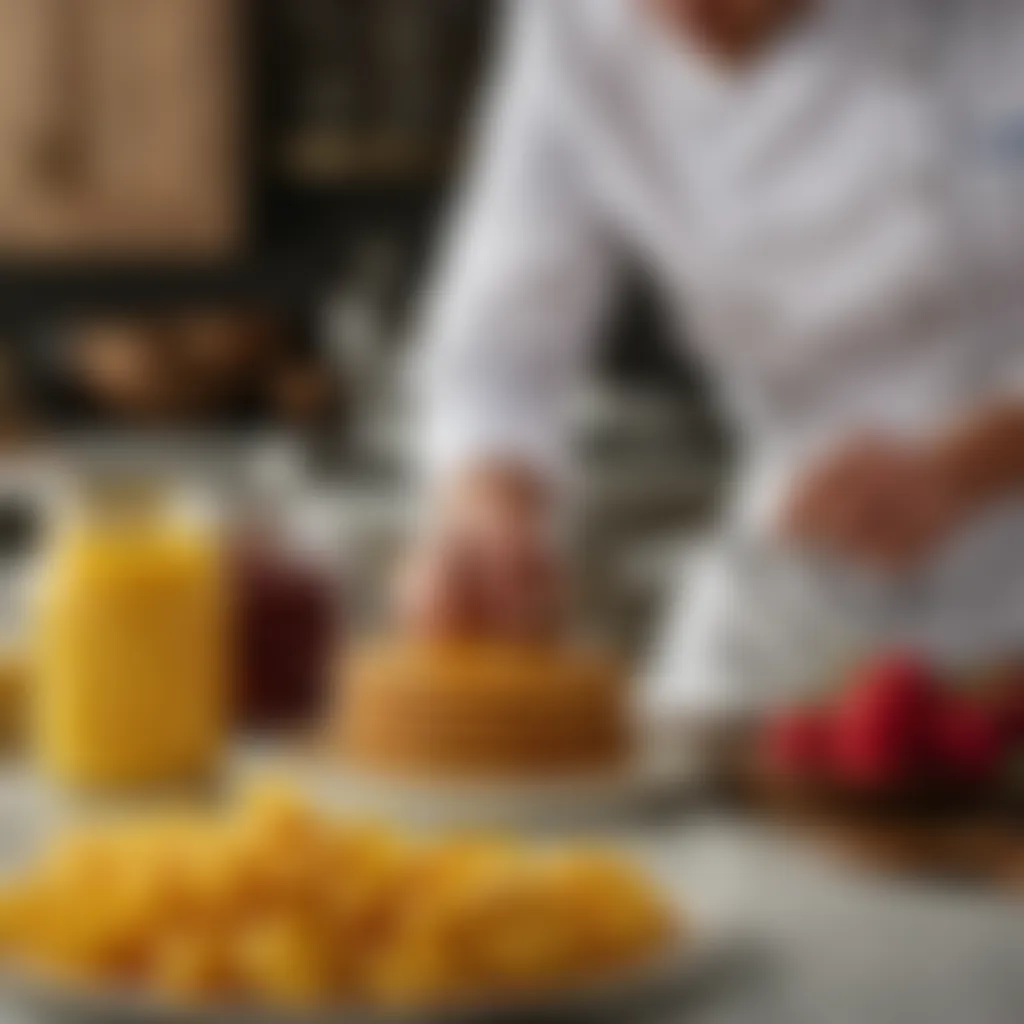
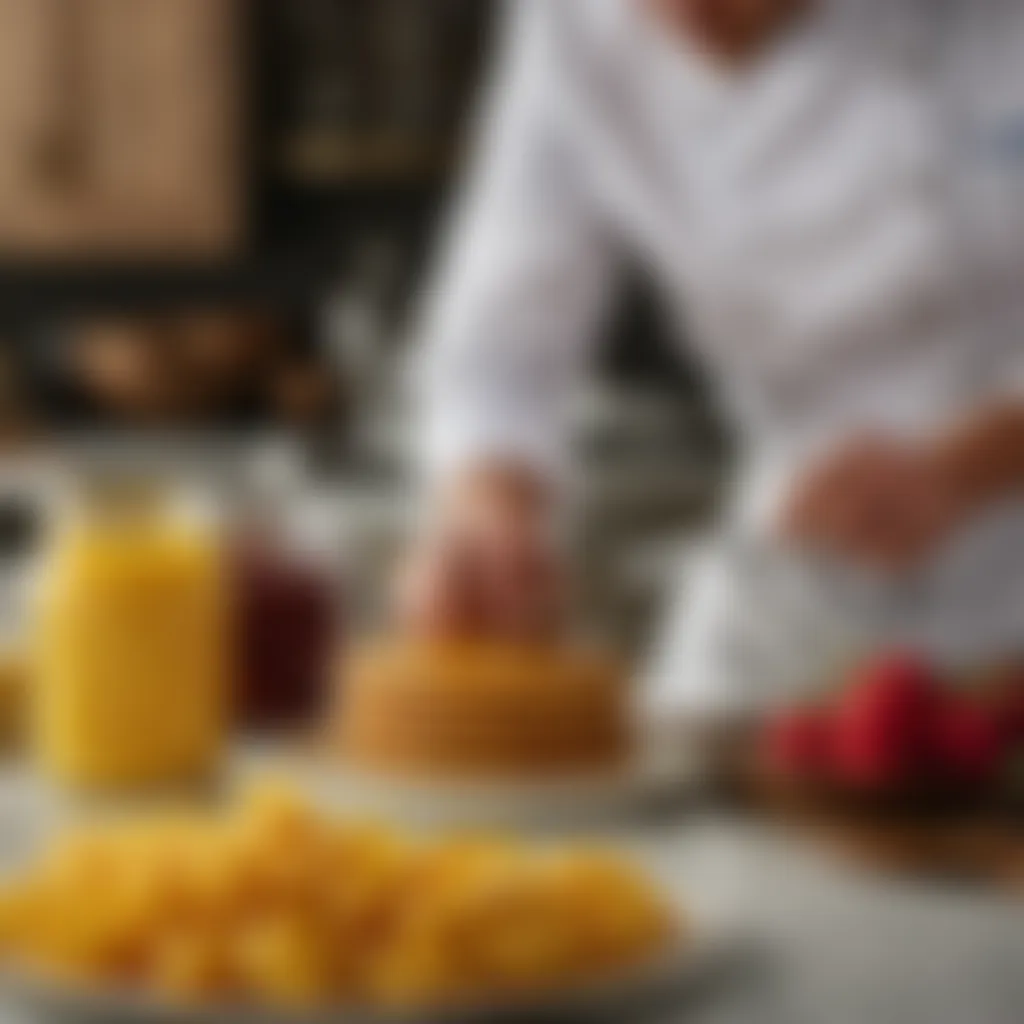
- Tomatoes and Olive Oil: This duo not only tastes great but also helps absorb lycopene, a powerful antioxidant, more effectively.
- Chili and Cumin: The warmth of cumin amplifies chili’s heat, creating a depth of flavor that resonates well in Mexican and Indian cuisines.
Housewives can experiment with combinations that might seem unusual at first, but often lead to new family favorites. For instance, who would have thought that chocolate and avocado could create a decadent dessert without the usual guilt?
This practice of pairing encourages creativity in the kitchen. When one learns to recognize potential combinations through ingredient analysis, meals become adventures rather than chores.
"The beauty of cooking lies in exploration; using data to enhance culinary practices turns every meal into an opportunity for innovation."
Regardless of skill level, everyone can benefit from understanding ingredient pairings—not only in meal preparation but also in optimizing flavors while ensuring nutritional balance.
Machine Learning in Recipe Analysis
Machine learning has made a considerable mark in dissecting the culinary world. When we talk about recipe datasets, the integration of this technology opens doors that allow data analysts to uncover patterns, trends, and even predict future food preferences. This isn’t just a novelty; it’s the bridge that connects raw data with actionable insights that can shape how we approach cooking and eating.
Understanding machine learning in recipe analysis hinges on identifying specific elements that make this technology beneficial when analyzing culinary data. For one, it enhances the personalization of culinary experiences. With predictive algorithms, it’s possible to recommend recipes based on an individual’s previous cooking habits or dietary restrictions. Imagine getting suggestions for dinner that consider not just what you’ve made before, but also your nutritional goals and even what ’s in your pantry. This practical application can save time and foster creativity in the kitchen.
Furthermore, the consideration of trends in dietary preferences, like plant-based or gluten-free diets, has never been more vital. As these trends shift, machine learning can adapt swiftly to provide recommendations that resonate with a changing market. For housewives or anyone keen on meal planning, being informed about these trends can enrich the quality and variety of meals being prepared at home.
These models also pave the way for companies to enhance product development, ensuring that new offerings meet consumer needs. By analyzing past data, businesses can predict which flavors or ingredients might hit the mark in upcoming cookbooks or food product lines. Thus, machine learning doesn’t just serve the curious home cook; it’s a powerful tool for the food industry at large, redefining how recipe datasets influence culinary choices.
Predictive Modeling
Predictive modeling plays a crucial role in utilizing recipe datasets effectively. In the context of cooking, this means using historical data to forecast trends in recipe preferences. A great example is analyzing user data from cooking platforms or recipe-sharing websites. By examining past user interactions, machine learning models can suggest recipes that users are likely to enjoy based on their prior choices.
- How does this work?
- Data is collected from various sources, including previous user submissions, ratings, and even social media trends.
- This data is cleaned, structured, and then fed into predictive algorithms.
- The model then learns which ingredients or cuisines are most appealing to specific demographics.
Housewives can particularly benefit from these models as they seek to diversify their cooking without straying far from what their family enjoys. For instance, if a predictive model indicates a rising interest in Mediterranean recipes among peers, a savvy cook could introduce those dishes to their family, tapping into a culinary trend that resonates.
"The ability to anticipate food preferences combines the art of cooking with the science of data analysis."
Clustering Recipes
Clustering recipes is another fascinating aspect of machine learning in culinary data analysis. Contrary to predictive modeling that focuses on forecasting, clustering groups similar recipes based on shared attributes. This approach can reveal hidden patterns in how dishes are categorized, be it through ingredient types, cooking methods, or even regional cuisines.
Imagine if you were organizing your cookbook or digital recipe folders. Clustering helps in identifying common threads among recipes, enabling the creation of themed meal plans, such as:
- Ingredient-Based Clusters: Recipes utilizing similar ingredients, great for minimizing waste.
- Cuisine Clusters: Grouping recipes from the same cuisine can lead to discovering new dishes within a familiar framework.
- Health Clusters: All healthier recipes can be bunched together, guiding meal planning for dietary needs.
The possibility of finding inspiration from dishes you would never have considered is a significant advantage of clustering. If you notice that many recipes featuring chickpeas can be grouped together, you might feel inspired to explore different ways of preparing them—thus expanding your cooking repertoire.
In summary, as the intersection of machine learning and culinary arts continues to grow, the use of predictive modeling and clustering allows for deeper insights into recipe datasets. The benefits extend beyond simple number-crunching; they create a more personalized cooking experience that resonates well with modern consumers, ensuring that home cooks feel empowered in the kitchen.
Applications of Recipe Datasets
Recipe datasets serve as a treasure trove of information, presenting diverse opportunities across various fields. This section discusses how such datasets are integral to product development and menu optimization—a definitive part of culinary innovation and consumer engagement.
Product Development
In the realm of food industry, product development is crucial. Recipe datasets hold the potential to inspire new food products by uncovering emerging flavors and ingredient combinations. Analyzing these datasets enables food scientists and culinary experts to see patterns that point toward consumer preferences. For instance, a dataset might reveal a rising trend in plant-based meals, prompting product developers to design innovative substitutes that cater to vegetarian and vegan customers.
The beauty of utilizing recipe datasets lies in their capability to provide a comprehensive view of how ingredients interact within dishes. This not only informs product innovation but also enhances recipe consistency and quality.
- Benefits of Recipe Analysis in Product Development:
- Insight into popular ingredients and flavors.
- Speed up the development and testing phase.
- Facilitation of nutritional analysis to meet health standards.
However, challenges persist, such as the need for maintaining data quality. Relying solely on datasets without robust validation may lead to misguided product launches.
Menu Optimization
Another vital application of recipe datasets lies in menu optimization for restaurants and food businesses. Utilizing analytics derived from these datasets can refine menu offerings, ensuring they align with customer desires and seasonal trends.
Personalized Recommendations
In today's competitive food market, personalized recommendations stand out as a game changer. When restaurants leverage recipe datasets, they can analyze dietary preferences, like gluten-free or low-carb meals, to suggest dishes that resonate with individual clientele. The specificity of such recommendations fosters customer loyalty and enhances the dining experience. Breaking each dish into constituent ingredients allows for a tailored approach; for example, if a diner loves Italian cuisine, systems can push effective recommendations based on their past orders combined with trending Italian recipes.
- Characteristics of Personalized Recommendations:
- Tailored based on individual tastes and previous orders.
- Incorporation of dietary restrictions and trends.
- Increased customer satisfaction and retention rates.
Despite their merits, personalized recommendations may sometimes miss the mark, especially if the underlying data is not exhaustive or current. Therefore, timely updates and usability testing are paramount.
Seasonal Adaptations
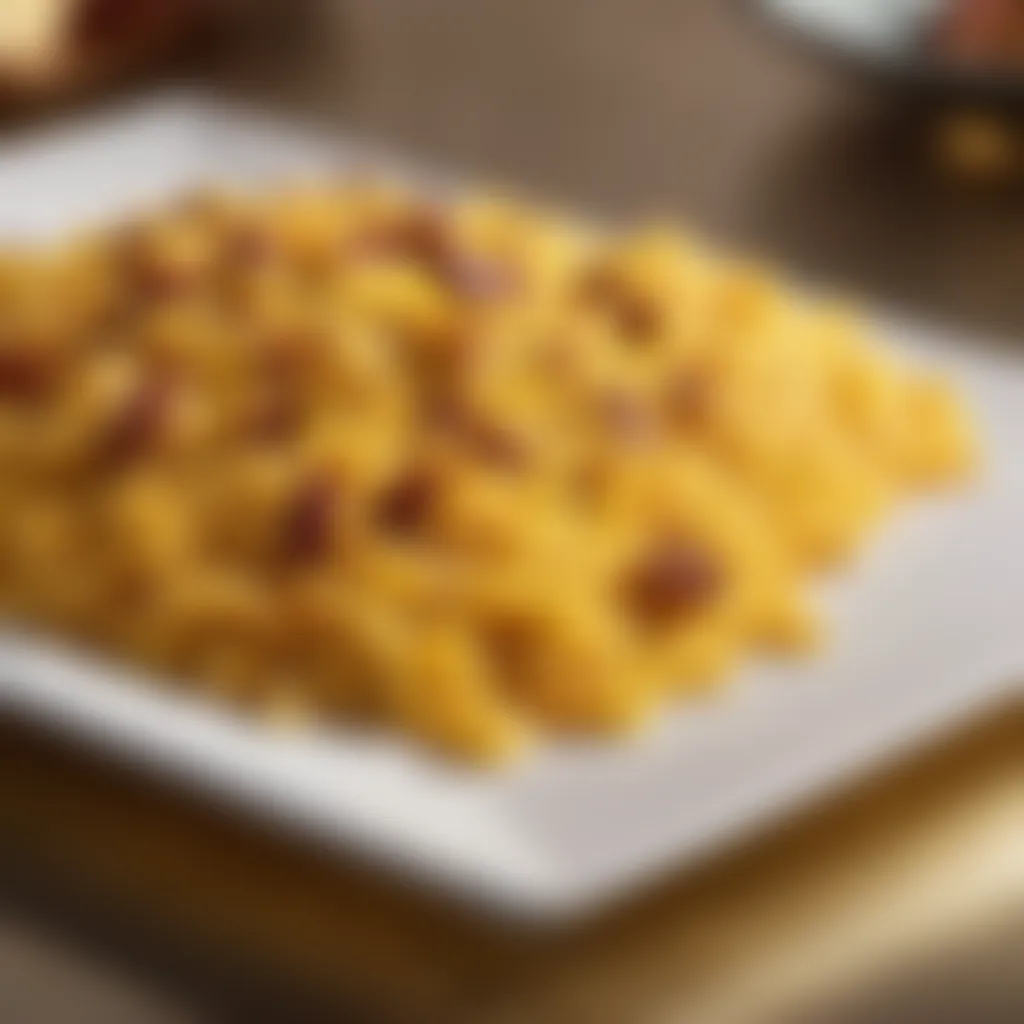
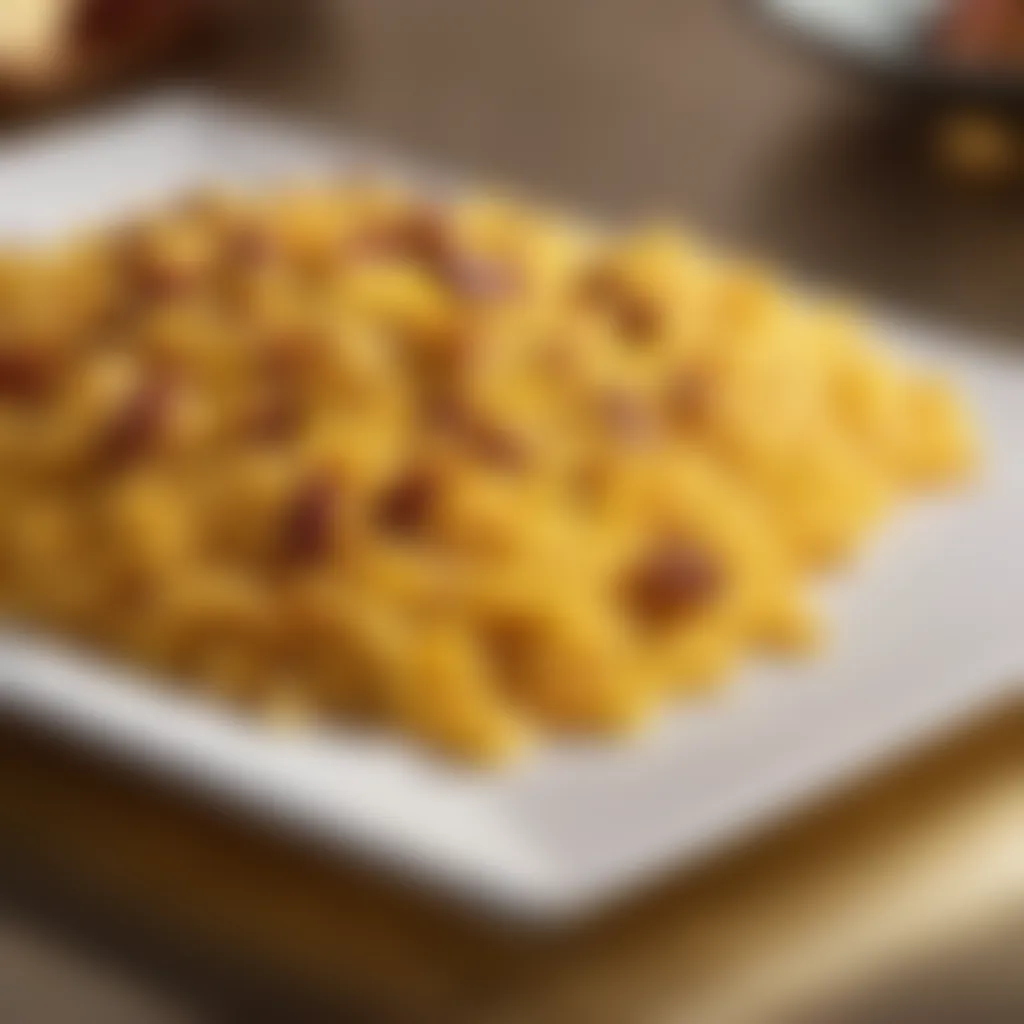
Seasonal adaptations revolve around optimizing menu items according to the changing seasons and ingredient availability. This concept revolves around using recipe datasets to forecast which dishes will be popular based on seasonal changes. For instance, during summer, there may be a surge in demand for lighter dishes and vibrant salads. By having access to a well-structured dataset, businesses can modify their menus to feature fresh and local ingredients, which not only appeals to customers but often comes with lower costs.
- Highlights of Seasonal Adaptations:
- Alignment with local produce availability, ensuring freshness.
- Enhanced appeal with seasonal marketing campaigns.
- Ability to draw customers who appreciate seasonal specialties.
Nevertheless, while adapting the menu based on seasons brings advantages, it necessitates careful consideration of customer preferences that aren’t solely dictated by time of year. It’s pivotal for businesses to maintain a balance between innovation and customer familiarity to ensure sustained interest in their offerings.
Recipe datasets pave the way for smarter decisions in culinary spaces, bridging the gap between consumer demands and product innovation while ensuring restaurant menus stay fresh and relevant.
Challenges in Utilizing Recipe Datasets
Understanding the challenges related to recipe datasets is as crucial as knowing how to use them. Various aspects come into play that can significantly affect the outcomes of any analysis. When trying to make sense of these datasets, it's important to be aware of issues that might impact the data quality and the cultural context. By addressing these challenges, one can better harness the power of recipe datasets to gain meaningful insights.
Data Quality Issues
Data quality is the backbone of any analysis—it dictates how trustworthy your findings can be. In the realm of recipe datasets, several problems can emerge that may skew the results.
- Inaccuracies in Data Entry: Recipes often vary in ingredients and amounts. A simple typo—like using "tablespoon" instead of "teaspoon"—can completely change the flavor profile of a dish.
- Inconsistent Measurements: Different recipes may use various units of measurement. Some may prefer metric while others utilize customary units. Without standardization, comparing data can feel like comparing apples and oranges.
- Missing Ingredients: Often, especially in user-generated content, some recipes leave out critical components, leading to an incomplete picture.
These issues can lead to faulty conclusions, and recognizing these imperfections is key to refining your analysis. Addressing them often requires cross-referencing multiple sources and employing robust cleaning methods. Without this diligence, the risk of ending up with misleading information is high, undermining the validity of culinary insights one might aim to extract.
"Data quality isn't just a technical issue; it's a culinary concern that can impact a chef's execution in the kitchen."
Cultural Context and Variability
Recipes are deeply embedded in cultural contexts, making it essential to consider this variability when analyzing datasets. People cook differently based on regional influences, traditions, and personal preferences.
- Regional Differences: What’s considered a standard ingredient in one culture might be a rare find in another. For instance, lemongrass is a common component in Southeast Asian cooking but could baffle a European cook.
- Traditional vs. Modern Recipes: While some datasets might focus on historical recipes, others may prioritize trendy, modern dishes. The contrast can introduce biases in data analysis, leading to skewed results.
- Personal Interpretation: Recipes often come with a degree of subjectivity. Each cook might alter ingredients to suit personal tastes or dietary restrictions, resulting in variations that can complicate analysis.
Understanding these cultural nuances is vital for interpreting recipe datasets accurately. It not only influences how recipes are created but also informs the preferences and trends in culinary consumption. Thus, appreciating the diversity of cooking styles can lead to richer and more nuanced insights from recipe data.
Future Directions in Recipe Data Analysis
Exploring the future directions in recipe data analysis is not just a venture into the unknown; it’s about tapping into the full potential of vast culinary knowledge trapped within datasets. As we look ahead, the importance of this topic becomes increasingly evident, touching on numerous aspects that promise to elevate culinary studies. Harnessing recipe datasets for expansive applications can lead to transformative insights that reshape how we approach not only cooking but also food science, nutrition, and cultural studies.
- Innovation in Food Technology: Emblematic of the cutting-edge advancements, the role of technology will continue to revolutionize data analysis in culinary fields.
- Cross-Pollination of Disciplines: Integrating recipe datasets with diverse fields will yield robust insights that can translate to better cooking practices and health standards.
- Consumer Behavior Insights: Understanding preferences and patterns will be paramount as datasets evolve, allowing for more personalized culinary experiences.
As we stand on the precipice, the possibilities seem endless, demanding our curiosity and willingness to adapt.
Technological Advancements
The rapid development of technology shapes every sector, and recipe data analysis is no exception. The significance of technological advancements lies in their capacity to improve data collection, analysis, and application processes. From sophisticated algorithms to artificial intelligence, the tools at our disposal are more powerful than ever.
For instance, machine learning can analyze hundreds of thousands of recipes to identify trending flavors or emerging dietary restrictions. This information can help chefs innovate and cater to evolving consumer needs. Additionally, sentiment analysis tools examine social media and culinary forums to gauge public opinion about certain dishes or trends.
Moreover, many new tools now enable home cooks and food enthusiasts to visualize data effectively. The emergence of visual cooking guides combines traditional knowledge with modern data interpretation, making it easier for everyone to grasp the importance of ingredients, cooking times, and techniques.
"The intersection of cuisine and technology is where culinary art meets science, creating infinite possibilities for creativity and understanding."
Integration with Other Datasets
The integration of recipe datasets with other types of data marks a pivotal shift in culinary analysis. This approach enables a multidimensional examination of foods and meals. By merging nutritional information, consumer behavior data, and agricultural statistics, we can glean comprehensive insights about food trends and public health.
For example, when recipe data intersects with agricultural trends, it can spotlight seasonal ingredients, promoting local sourcing and sustainability. This synergy not only supports eco-friendliness but also engages consumers in more mindful cooking practices.
Furthermore, by connecting recipes with demographic data, businesses can tailor products to address various cultural preferences while meeting dietary needs. This means a more inclusive culinary landscape where everyone’s voice is heard.
- Enhanced Personalization: Any integration paves the way for more custom recipe recommendations.
- Marketing Intelligence: Businesses gain access to nuanced consumer insights, driving better decision-making.
- Cultural Significance: Understanding historical context can enrich culinary experiences.
Thus, the future of recipe data analysis shines brightly when we consider these integrations. The interplay between various datasets promises not just to enhance our understanding of food but also to foster a sense of community among individuals with shared culinary interests.
The End
In concluding our exploration into recipe datasets and their significance in data analysis, it becomes clear that recipe datasets are not just collections of ingredients and instructions; they are invaluable repositories of knowledge that hold the potential to transform culinary practices and enhance the understanding of food culture.
Summarizing Key Insights
The weighty benefits offered by these datasets touch on several fundamental areas:
- Culinary Trends: By analyzing patterns in ingredients and preparation methods, we can track evolving tastes, accommodating dietary restrictions, and shifting consumer preferences.
- Nutritional Information: Detailed insights into the nutritional composition of recipes empower healthier cooking choices, promoting wellness among families. This can also pave the way for innovative product developments.
- Cultural Context: Recipes are steeped in cultural narratives. Understanding the data allows us to appreciate the varied backgrounds and traditions that influence what we eat.
- Data Quality Improvement: As discussed, challenges like data quality issues necessitate stringent control measures to deepen the reliability of insights derived from these datasets. Addressing this leads to more accurate analyses and robust applications in the food industry.
"Recipe datasets serve as a bridge connecting the art of cooking with the science of data analysis."
Each of these insights underscores that the effective use of recipe datasets can significantly contribute to various sectors—ranging from health and nutrition to culinary innovation, offering a win-win scenario for both consumers and businesses alike.
The Future of Culinary Data Analysis
Peering into the future, culinary data analysis is poised for a remarkable expansion. As artificial intelligence and machine learning technologies enhance our analytical capabilities, we can anticipate a few significant advancements:
- Personalization: The development of algorithms that tailor recipes to individual preferences and dietary needs will offer home cooks bespoke solutions, turning meal planning into a delight rather than a chore.
- Integration Across Platforms: Combining recipe datasets with retail, nutrition, and health datasets will yield comprehensive insights. For example, linking recipes to local ingredient availability strengthens community ties and supports local economies.
- Sustainability Focus: As awareness of environmental issues grows, recipe datasets can help analyze and promote sustainable cooking practices. This involves advocating for the use of local and seasonal produce, ultimately reducing food waste and carbon footprints.
- Increased Accessibility: Open-source databases and collaborative platforms will further democratize access to recipe datasets, empowering more people to engage in cooking and food preparation.
With these prospects in mind, the continued importance of recipe datasets in shaping future culinary landscapes cannot be overstated, making them a cornerstone of both tradition and innovation.