Mastering MLflow Integration for Machine Learning
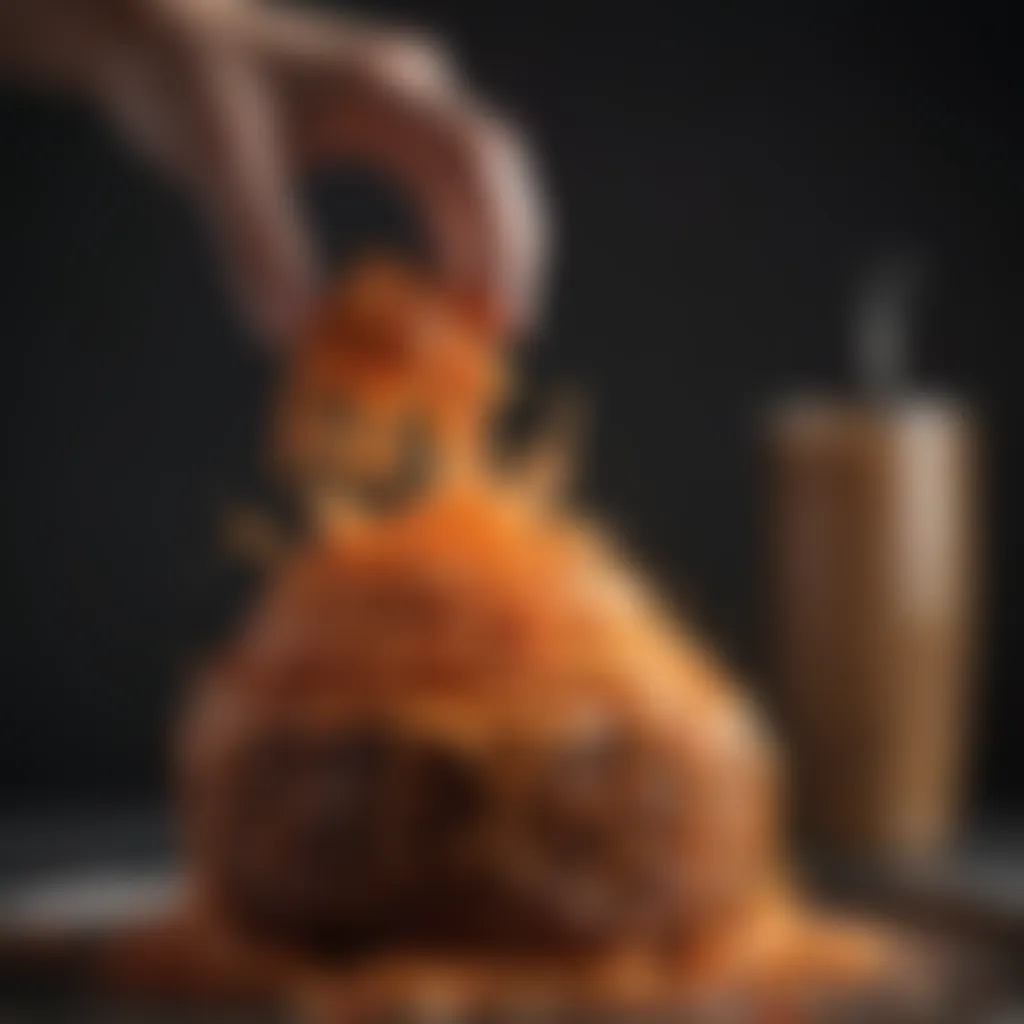
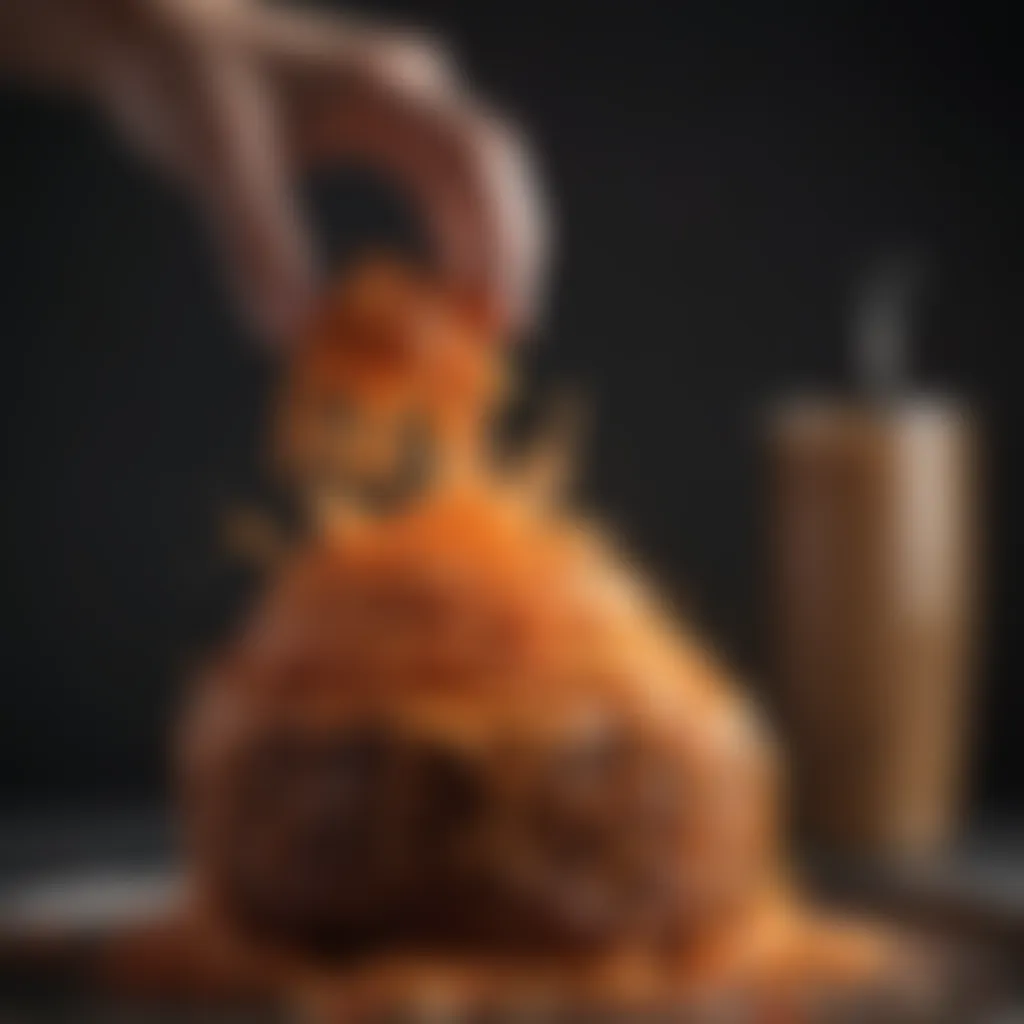
Intro
In the world of machine learning, having a robust system for managing experiments is not just beneficial; itâs essential. MLflow comes into play as an innovative platform that streamlines various aspects of the machine learning lifecycle. With its user-friendly interface and powerful functionalities, MLflow can significantly enhance your machine learning projects.
This guide aims to shed light on the nuts and bolts of integrating MLflow within your existing workflows. We'll break down the different components, architecture, and best practices, making sure you have a solid roadmap to follow. A deep dive into common challenges will also be included, offering practical solutions and enlightening case studies. With this knowledge, youâll be set to improve tracking, reproducibility, and collaboration in your ML endeavors.
Preparing your workspace for MLflow integration resembles what a deft housewife does while organizing her kitchen. Gathering the right ingredients sets the stage for seamless execution. Letâs begin our exploration with the essential components needed for successful MLflow integration.
Ingredients:
- MLflow Installation:
- Database:
- Cloud Services:
- Libraries and Tools:
- Python 3.6 or above
- Pip package manager
- SQLite for local storage
- PostgreSQL for a more robust solution
- AWS S3 or Azure Blob Storage for model storage
- Scikit-learn
- Pandas
- NumPy
Preparation Steps:
Having listed our ingredients, it's time to prepare. Hereâs a step-by-step process:
- Install MLflow: Open your terminal and run:
- Set Up the Environment: Depending on your needs, set environment variables. For example, for PostgreSQL, you might use:
- Configure Storage: Ensure you have a backend store ready for tracking and a location set for saving models.
Technical Aspects:
When it comes to technical specifications, getting it right is paramount. Here are some details to consider:
- Database Settings:
- Storage Configurations:
- For development, SQLite may suffice. But PostgreSQL handles larger workloads better.
- When using AWS S3, ensure you have the right permissions and keys set up properly.
- Use to set your storage option in code.
Cooking Process:
Now that the preparation is underway, let's look at the sequential steps:
- Track Experiments: Use to begin tracking. This is like lighting the stove; ignition is key.
- Log Parameters and Metrics: As your model trains, log parameters with , and metrics via . Documentation looks like:
- Save Models: Once satisfied with your model, save it using , ensuring reproducibility.
Troubleshooting Tips:
Even experienced cooks run into trouble. Here are some common pitfalls:
- Model Not saving?
Verify your storage permissions and configurations. Missing credentials will stop you cold. - Experiments not logging?
Check the URI setup. If it's incorrect, nothingâs getting saved.
Remember: A solid foundation is pivotal. Just like in baking, the right measurements yield better results.
Prolusion to MLflow
In the realm of machine learning, keeping track of models, their versions, and associated data can feel like herding cats. Managing these elements effectively leads to smoother workflows and more successful outcomes. Thatâs where MLflow comes into play. Itâs designed to serve as an essential toolset to streamline the entire machine learning lifecycle. This section serves as an entry point to understand why MLflow is crucial for anyone dabbling in or deeply immersed within the complexities of machine learning.
Defining MLflow
First things first, let's get our heads wrapped around what MLflow actually is. At its core, MLflow is an open-source platform primarily focused on managing the machine learning lifecycle. Think of it as a Swiss army knife for your ML projects, providing utilities for tracking experiments, packaging code into reproducible runs, and managing and deploying models.
MLflow consists of four main components:
- MLflow Tracking: Keep tabs on your experimentation. You can log metrics, parameters, and artifacts all under one roof.
- MLflow Projects: Package your code in a way that anyone can run it using a standard format, enhancing collaboration.
- MLflow Models: Support for multiple deployment formats means youâll be better equipped to deploy your models wherever you need.
- MLflow Registry: This acts as a centralized place to manage your models, tracking versions and providing insights into transitions from development to production.
In brief, MLflow simplifies many complexities and helps ensure that all your hard work doesnât go up in smoke.
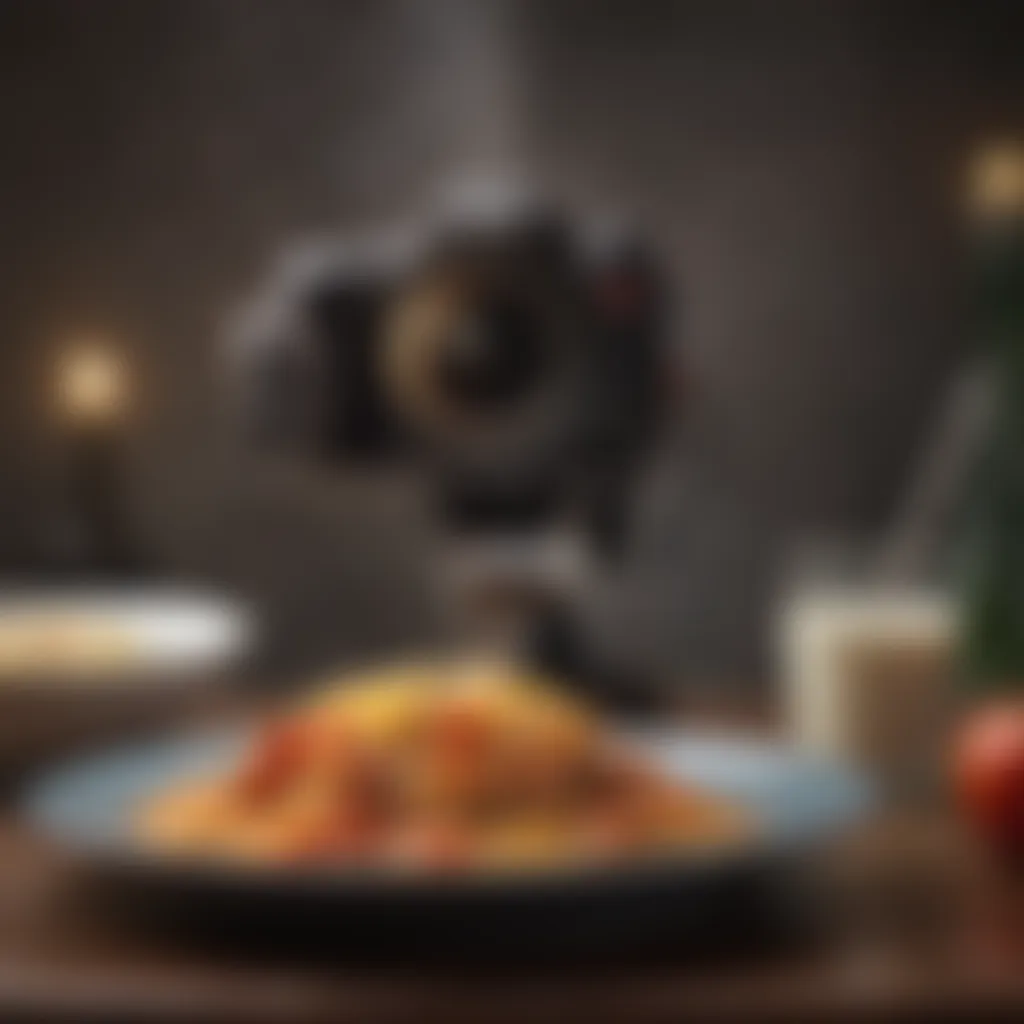
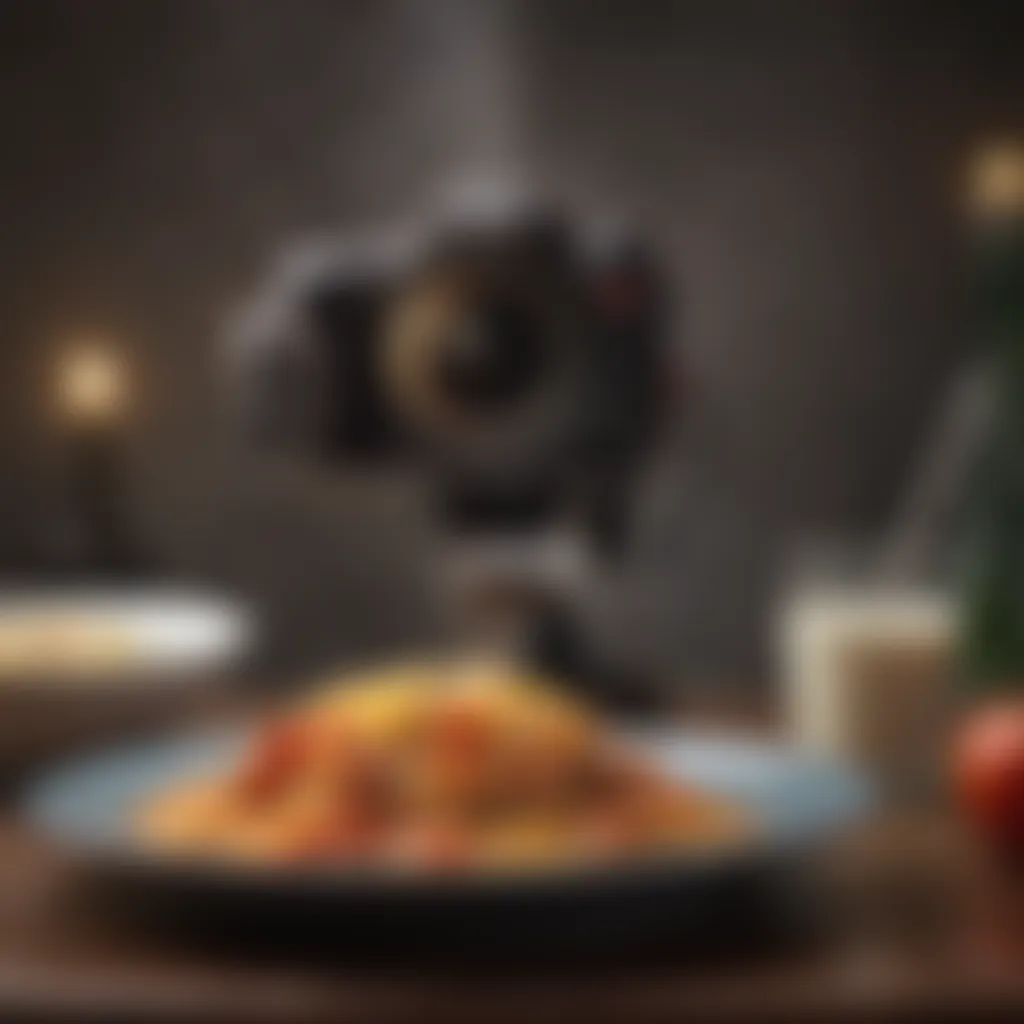
Importance of Tools
Now, why should you pay attention to tools like MLflow? Well, success in machine learning relies heavily on managing experiments effectively. Imagine spending hours tuning hyperparameters on various datasets, only to lose track of which settings worked best. Thatâs a recipe for productivity loss!
Having a robust tool to facilitate your work can lead to:
- Improved Collaboration: By utilizing standard formats, team members can easily work on projects together without stepping on each otherâs toes.
- Reproducibility: When you keep detailed logs of your experiments, you can go back and replicate or build on your findings, which is invaluable in a field driven by continuous learning.
- Time-Saving: Automating the tracking of metrics means less manual work and more time for actual data analysis.
"The time you save with ML tools can drastically improve the quality of your outcomes."
All in all, understanding and utilizing MLflow can save you from everyday pitfalls and headaches, making your machine learning journey smoother. As we delve deeper into this guide, youâll learn how to integrate MLflow into your existing workflows, explore its core components, and discover best practices for effective use.
Core Components of MLflow
Understanding the core components of MLflow is essential for anyone looking to effectively integrate this tool into their machine learning workflows. Each component serves a distinct purpose, contributing to the overall efficacy of your projects. The interplay of these elements can streamline your processes, enhance reproducibility, and facilitate collaboration. Letâs break down the key components that make MLflow a robust choice for managing machine learning life cycles.
MLflow Tracking
MLflow Tracking is the backbone of the MLflow system, providing a systematic way to log all aspects of your experiments. This component helps you track parameters, metrics, and artifacts, creating a narrative of your model's lifecycle.
Think of it as your kitchen notebook where you jot down the exact measurements, cooking times, and even the little tweaks you make as you experiment with recipes. In this analogy, your cooking experiment is akin to machine learning tasksâdetails matter greatly. By effectively using MLflow Tracking, data scientists can revisit earlier models, analyze what worked and what didnât, and refine their processes accordingly.
With other tools, it can be easy to lose track of how a particular model was built or which parameters produced the best results. MLflow Tracking keeps all this information organized and accessible, thus making the analysis seamless.
MLflow Projects
MLflow Projects introduce a standardized way to package your code in a reusable manner. A project can be viewed as a recipe card that not only contains the ingredients but also specifies the method of preparation. You can define the project using a file, making it easy to share with others or run on different systems.
Being able to encapsulate your code allows teams to collaborate effectively. Suppose you're part of a baking group; if everyone uses the same recipe but tweaks it a bit differently each time, you could end up with wildly different results. The same thing can happen in data science if your code isn't properly managed. With MLflow Projects, you keep your project's integrity intact, facilitating peer review and collaborative innovation.
MLflow Models
MLflow Models serve as a deployment interface for your machine learning models. This component enables you to manage multiple versions of your models and deploy them in a streamlined manner. Think of it as your go-to cookbook that not only lists the final recipes but also includes various adaptations for different dietary needs.
When models are easy to deploy, they can be integrated into applications swiftly. Whether you're serving a model as a REST API, or packaging it for cloud-based services, the versatility of MLflow Models simplifies the process significantly. The standardized format helps in managing various deployment stage, so you won't have to reinvent the wheel each time you move to production.
MLflow Registry
The MLflow Registry stands out as a model management feature that allows you to organize and control different versions of your models. Imagine a well-organized pantry, where similar ingredients are grouped together, and you can easily select the one you need. The Registry provides structure and visibility over your models, helping maintain quality and compliance.
This component offers functionalities such as versioning, stage transitions, and annotations, enabling teams to keep track of changes and ensure that they always have access to the most relevant models. You can push a model from âStagingâ to âProductionâ simply and maintain control over each version, much like you would manage which dishes are served from a menu based on seasonal availability.
In summary, the core components of MLflow work in unison to provide a comprehensive solution for managing machine learning workflows. By effectively utilizing MLflow Tracking, Projects, Models, and the Registry, teams can better manage their machine learning processes, ultimately leading to impactful results.
Setting Up MLflow
Setting up MLflow is like laying the foundation of a house; it forms the base upon which all future structures are built. If you want to take advantage of MLflow's powerful features, proper setup is crucial. Without it, you might find yourself grappling with issues that could hinder your machine learning projects' progress. Moreover, once you grasp the significance of setting up MLflow correctly, youâll appreciate the benefits it brings, such as streamlined workflows, improved collaboration among team members, and much better tracking of experiments.
Installation Process
Installing MLflow is the first step in your journey. If you think itâs just an ordinary task, think again! The right installation process will save you a ton of headaches down the line.
To get started, you will need to ensure that you have the proper environment. MLflow works seamlessly with Python, so having a recent version installed on your machine is essential. Below are the steps to install MLflow:
- Open your terminal or command prompt.
- Create a virtual environment (optional but recommended):
- Install MLflow using pip:
- Verifying the installation: After installing, you can check if MLflow is installed correctly by typing:
These steps should set you up for success. Itâs a bit like setting up your kitchen before cooking; you want everything in its place. Once youâve installed MLflow, youâll find itâs more straightforward than baking a pie!
Configuration Requirements
After installation, the next logical step involves configuring MLflow to suit your needs. This aspect is just as important as the installation itself. You wouldnât start driving without adjusting your seat or mirrors, right?
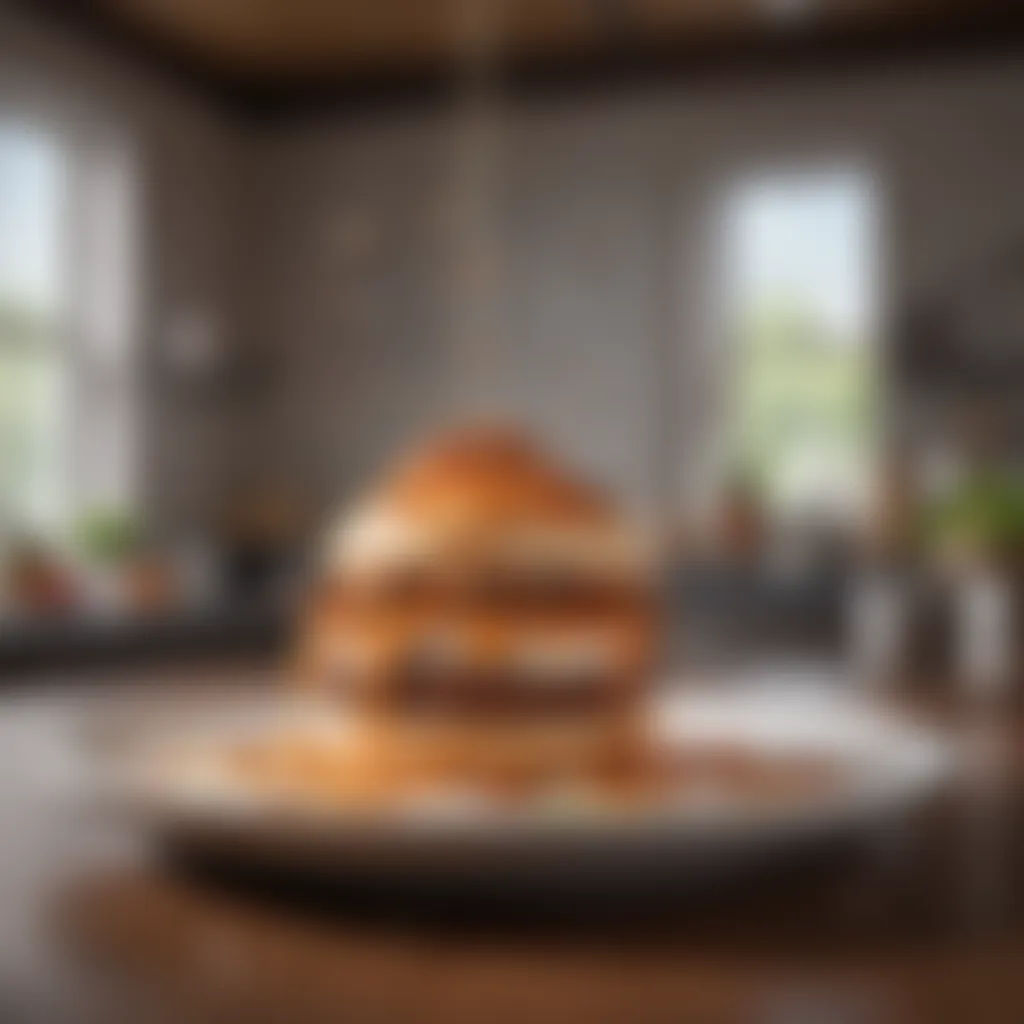
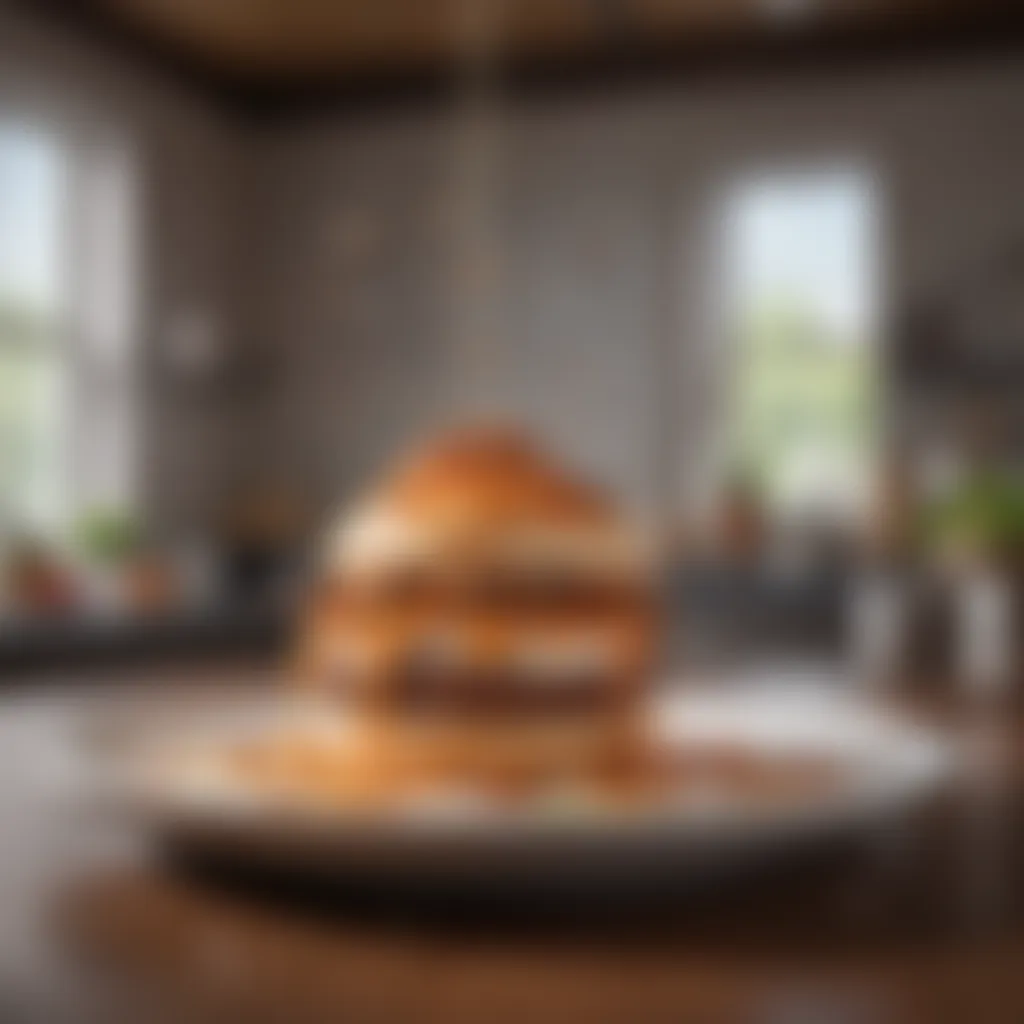
Several configurations allow MLflow to function optimally. Hereâs what you need to keep in mind:
- Backend Store: MLflow needs a backend store to log your metrics, parameters, and artifacts. You can use a local filesystem for testing, but for production, consider using databases like PostgreSQL or MySQL for reliability.
- Artifact Store: Just like a baker wouldnât store cookies on the floor, you need to choose where to save artifacts like models and plots. Options include local storage, AWS S3, Azure Blob Storage, or Google Cloud Storage, depending on your setup and preferences.
- MLflow Tracking URI: This is the location where MLflowâs tracking server will log an experiment. It can be set to a URI if you decide to use a centralized tracking server. Itâs vital to do this to keep all experiment data in one accessible place.
Important: Always be aware of your data privacy and security when configuring your backend and artifact stores, especially while dealing with sensitive or proprietary information.
With these configurations in place, you can think of all your machine learning workflows as neatly organized â akin to a pantry stocked with ingredients for all your favorite dishes. Youâll be ready to dive into the actual integration and make the most of what MLflow offers!
No matter if youâre a beginner or a seasoned pro, understanding the essentials of setting up MLflow will pave the way for smoother sailing ahead.
Integrating MLflow into Existing Workflows
In the fast-paced world of machine learning, where data flows like a river after heavy rains, integrating tools like MLflow into existing workflows is not merely a suggestion. Itâs an essential step that can spell the difference between chaos or streamlined success. When you think about it, MLflow offers frameworks for tracking, organizing, and sharing experiments, which can be a boon for current processes. This section delves into the crucial facets of this integration and why effectively fusing MLflow into your existing workflows can transform your entire machine learning operation.
Assessing Workflow Needs
Understanding the specific requirements of your machine learning workflows forms the backbone of successful integration of MLflow. Before you leap headfirst into merging MLflow with your operations, itâs wise to take a step back and evaluate the nuances of your current setup.
- Identify Key Stakeholders: Start by gathering input from everyone involved. This includes data scientists, engineers, and even end-users who may not be directly related to ML projects. Their insights can guide how MLflow can best serve the workflow.
- Document Current Processes: Get a clear picture of the existing workflow. What processes take place, and what are the pain points? If someoneâs pulling their hair out because of manual tracking, thatâs a sign MLflow could help.
- Determine Integration Goals: Establish what you aim to achieve through this integration. Is it better tracking of experiments, improved organization, or a smoother collaboration among team members?
- Evaluate Infrastructure Compatibility: Check whether your existing systemsâbe it databases, cloud services, or local setupsâare compatible with MLflow. This compatibility is vital for a seamless transition.
Every small detail counts. Take the time to consider how MLflow will fit into your unique puzzle. After all, having a hammer doesnât necessarily mean you should be building a house if the foundation isnât set right.
Modifying Data Pipelines
Once youâve assessed your workflow needs, it's time to pivot focus towards modifying your data pipelines. This step might feel daunting, but think of it as fine-tuning an instrument; it can lead to harmonious outcomes. Hereâs how to approach this crucial task:
- Integrate with Data Sources: Whether you are using databases like MySQL or platforms like AWS S3, ensure that MLflow can tap into these data sources easily. Establishing connections is key to facilitating smooth data flow.
- Design Workflow for Experiment Tracking: Modify existing data pipelines to embed MLflowâs tracking capabilities. This may involve adjusting how data is logged or reported during experiments. You want to be able to track metrics and parameters in a way that aligns with your specific objectives.
- Automate Data Ingestion: Create mechanisms for real-time or batch data ingestion as per your needs. Automating this can save valuable time and minimize errors, letting you focus more on analyzing results rather than wrestling with data retrieval.
- Consider Data Quality Checks: Introducing MLflow means that you're likely collecting a heap of data. Ensure that you embed data quality checks within your pipeline. This will prevent skewed results, making your ML endeavor more reliable.
Integrating MLflow into your workflows isnât just a technical feat; itâs a strategic move aimed at efficiency and effectiveness in the vast landscape of machine learning.
By thoughtfully assessing your workflow needs and tweaking your data pipelines, the integration of MLflow can occur seamlessly, allowing you to tap into its full potential. The transition might require effort upfront, but the long-term gains will more than make up for it.
Best Practices for MLflow Usage
When it comes to working with MLflow, following best practices ensures that your machine learning workflows are not just functional but also efficient and effective. These practices serve as the backbone for utilizing MLflowâs capabilities fully, allowing you to manage experiments, track models, and facilitate collaboration among teams. By implementing these principles, you can significantly enhance the reproducibility and reliability of your machine learning projects.
Version Control for Experiments
A critical aspect of engaging with MLflow is employing effective version control for your experiments. When you conduct experiments, each run generates its own set of parameters, metrics, and artifacts. Keeping a tidy record of these variations can quickly turn chaotic without a strong version control strategy.
By leveraging MLflowâs built-in capabilities, which allow for tagging different versions of both datasets and models, you maintain clarity over project evolution. Think of it this way: imagine trying to find a favorite recipe among a stack of handwritten notes with no dating or versioning; youâd be hard-pressed to recreate exactly what worked. Thatâs where version control shines. Although it may feel tedious at first, establishing this practice leads to significant benefits:
- Easier Comparisons: You can steadfastly revisit any previous experiment, analyze what went right or wrong, and refine your approach accordingly.
- Traceability: In a world where regulations on data and its use are tightening, being able to trace back through versions can help you comply with necessary guidelines.
- Collaborative Efforts: If multiple folks are working on the same project, you minimize the risk of overwriting each otherâs work, keeping everyone on the same page.
To illustrate this, consider a situation where a data scientist iteratively tests various algorithms on the same dataset. With proper versioning, they can return to the most successful variant without the headache of sifting through countless revisions.
Creating Reproducible Workflows
Creating reproducible workflows involves more than ensuring that your code runs without errors; itâs about establishing a systematic method that can be repeated with the same outcomes, even by someone else. MLflow supports this by allowing you to capture the entire lifecycle of a machine learning model, from the initial experiment to deployment.
Here are some key elements to focus on to develop a reproducible workflow:
- Utilize MLflow Projects: They organize all aspects of your model, from code to environment specifications. Packaging your projects means anyone can clone them, run them, and expect consistent results.
- Specify Environments: Use files to specify your libraries and dependencies. This way, when someone else tries to run your project, they wonât face compatibility problems due to missing packages or different library versions.
- Document Your Process: Never underestimate the power of good documentation. Write down what worked, what didnât, and why you chose a particular approach. This isnât just for others; it can help jog your memory when you come back to the project after some time.
- Automate Reporting: Automating the creation of reports that showcase experiment results and comparisons fosters consistency. MLflowâs capabilities support this, allowing you to generate visualizations and track metrics seamlessly.
"Reproducibility is the bedrock for any scientific inquiry. It elevates the quality of findings and fosters trust in the results."
By weaving these threads into your MLflow usage, you can transform your machine learning projects from disarray into systematic, reproducible workflows. After all, the goal is to ensure that youâre not just cooking up solutions but serving a dish that everyone can follow and replicate with ease.
Common Challenges in MLflow Integration
Integrating MLflow into machine learning workflows is not just a hop, skip, and jump. In fact, many practitioners find themselves stumbling on the bumpy road of integration. This section aims to shed light on common challenges that can arise during the integration process, emphasizing the significance of addressing these issues. As machine learning becomes more prevalent, itâs essential to recognize these challenges so that teams can find solutions that enhance their workflows. Understanding the problems can pave the way for a smoother ride, ultimately ensuring that the power of MLflow is harnessed effectively.
Navigating Technical Hurdles
When diving into MLflow, itâs easy to run into technical obstacles that can feel like a brick wall. Usually, these hurdles come from configuration mismatches, complex environment setups, or lack of understanding of MLflowâs components. Here are some common issues that teams may face:
- Dependency Conflicts: Different projects might require specific versions of libraries. These conflicts can cause confusion and lead to integration failures.
- Configuration Errors: A simple misconfiguration in the MLflow setup can hinder tracking or model deployment effectively. Every little parameter matters.
- Compatibility Issues: Integrating existing tools and platforms with MLflow can be tricky. The lack of compatibility might require additional workarounds or modifications.
To mitigate these challenges, having a solid foundational understanding of both the technology and the integrated systems is key. Furthermore, thorough documentation and version controls can alleviate many of these technical hurdles. By implementing regular checks and reviews, teams can quickly identify what's holding them back.
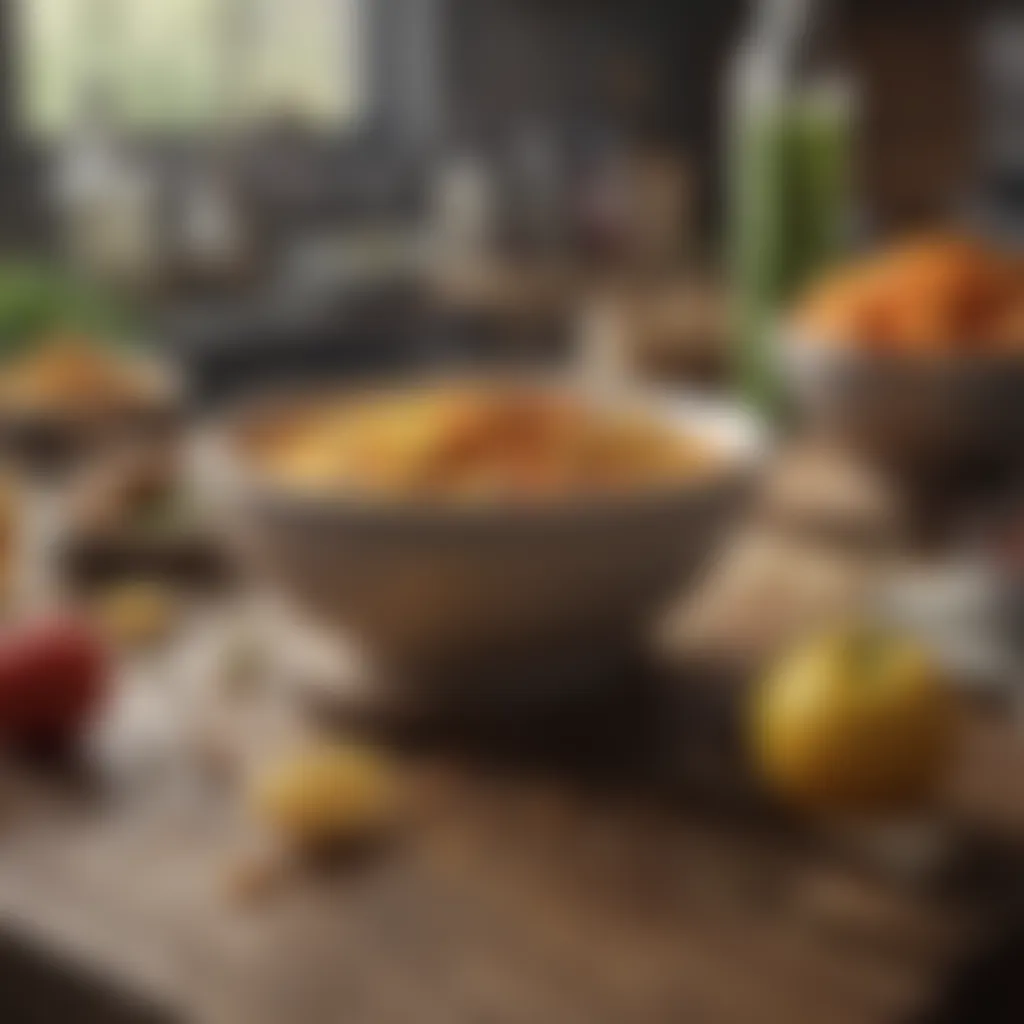
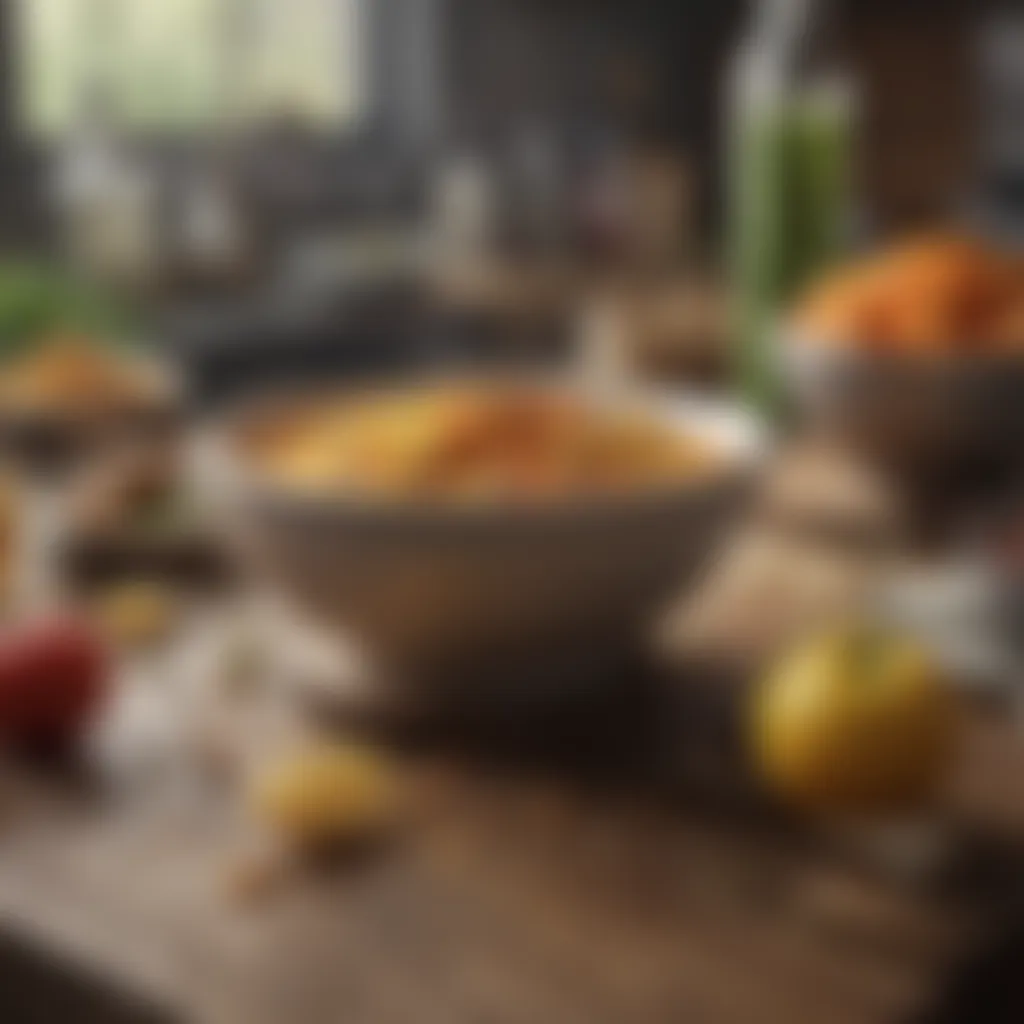
Data Management Difficulties
Data management is often the Achilles' heel for many projects, especially when MLflow comes into play. Without proper data management, the entire integration could feel like trying to build a house on sand. Common data management pitfalls include:
- Data Inconsistency: When datasets vary in structure or format across different stages of the workflow, it can lead to errors and uncertainties.
- Version Control for Data: Just like code, data too can evolve. Tracking changes in datasets is crucial, yet many teams overlook this aspect until it's too late.
- Storage Issues: Managing storage efficiently is another sticky point. Different types of data require appropriate storage solutions, and using the wrong type can incur performance hits.
Addressing data management difficulties involves creating systematic practices for data organization and versioning. Leveraging tools designed for data tracking and ensuring proper data formats can save time and hassle down the line. A solid data management plan is like a sturdy foundation; it supports everything built upon it.
"A stitch in time saves nine." Therefore, tackling these challenges at the onset can spare teams from a myriad of headaches later.
In summary, being aware of the common challenges faced during MLflow integration is the first step towards successful implementation. By proactively addressing technical risks and data management pitfalls, users can better position themselves to reap the benefits of MLflow, leading to improved tracking and model management.
Case Studies in Successful Integration
Understanding practical applications of MLflow integration enhances our comprehension of its real-world effectiveness. By assessing case studies from various sectors, we see not just theory but real outcomes. These examples illustrate challenges and resolutions that are key to navigating the complex landscape of machine learning workflows. They also showcase how MLflow can provide tangible benefits across different scalesâ be it in a vast enterprise environment or within the nimble infrastructure of a small business.
"Real-world applications provide context that no theory can match; they are the litmus test for any toolâs effectiveness."
Enterprise Solutions
In large organizations, the stakes are higher, and often the complexity is off the charts. Take, for instance, a leading financial institution that integrated MLflow into its fraud detection system. This institution faced an enormous amount of data daily, making it imperative to streamline operations involving model tracking and deployment.
By utilizing MLflow's tracking component, the team was able to automatically log each experiment, capturing parameters, metrics, and output in a centralized place. This meant that data scientists could easily navigate through numerous iterations of models and refine their strategies toward better accuracy.
Hereâs what stood out:
- Clear Versioning: Each model version was easily retrievable, allowing for rapid rollbacks if a newly deployed model underperformed.
- Team Collaboration: Different teams worked concurrently without stepping on each otherâs toes as MLflow allowed them to track the development process transparently.
- Automation: Automated logging eliminated the tedious manual process, thus enabling the data scientists to focus more on developing innovative algorithms instead of working on administrative tasks.
In their case, the benefits were apparentâ improved accuracy in detecting fraudulent transactions and significant reductions in operational overhead.
Small Business Applications
Now, letâs look at a local bakery that also took the plunge with MLflow. Their goal? To optimize inventory management based on predictive analytics. With a smaller tech team, they needed something that was both powerful and simple to manage. They introduced MLflow to monitor order patterns and forecast demand, replacing guesswork with data-driven decisions.
Hereâs how this small-scale integration worked:
- Simplicity of Setup: The bakery found the installation straightforward, which was crucial given the limited technical resources.
- Collaborative Efforts: By tracking models, the staff could analyze what sold best on specific days, leading to smarter purchasing and stock decisions.
- Adaptable Models: With fluctuating customer preferences, MLflow helped them regularly update their models. This made adjustments seamless rather than a disruptive process.
In this scenario, the bakery not only improved its efficiency but also curtailed wasteâ ultimately a win-win situation.
The case studies from both enterprise and small business contexts underline the versatility and utility of MLflow. These narratives serve to guide other organizationsâlarge or smallâ in applying MLflow effectively, underscoring the importance of adaptation and practical implementation in successful integrations.
Future of MLflow
Looking ahead, the future of MLflow is as intriguing as a well-crafted out-of-the-oven pie, offering a blend of opportunities and challenges. As the machine learning landscape evolves, MLflow stands poised to adapt, ensuring that practitioners can navigate this ever-changing terrain with ease. In this section, we will delve into the emerging trends shaping its trajectory and contemplate potential updates and improvements that can enhance its efficacy.
Emerging Trends
The momentum surrounding MLflow is not just a passing fad; it's driven by several key trends that signal a promising future. Notably, the increasing push for automation in machine learning operations (MLOps) is one significant element. As firms look to streamline their processes, MLflow is being integrated into automated frameworks.
- Integration with Cloud Services: As businesses migrate to cloud computing, MLflow will likely enhance its compatibility with major providers like Amazon Web Services and Google Cloud Platform. This shift allows for more accessible resource allocation based on workload demands.
- Expand Models Repository: There's a palpable buzz about the development of model repositories. As practitioners seek to share knowledge and leverage community expertise, these repositories will be pivotal. Expect updates that facilitate easier access and contributions from users around the globe.
- Enhanced Collaboration Features: As more teams transition to remote work, collaboration tools in MLflow will become essential. Feature updates that foster seamless communication among team members will be vital in enhancing productivity.
These trends not only create a more user-friendly experience but also ensure that MLflow remains relevant, helping practitioners address the specific needs of todayâs fast-paced environment.
Potential Updates and Improvements
As any seasoned cook knows, sometimes tweaks in a recipe can make all the difference. Similarly, MLflow can benefit from updates that refine its capabilities. Here are some enhancements to watch for:
- Expanded Integrations: The rise of numerous machine learning tools has led to a diverse ecosystem. Future versions of MLflow could include even broader integrations with popular libraries like TensorFlow and PyTorch, simplifying the workflow for users.
- User Experience Overhaul: Enhancing the user interface is crucial. A more intuitive design can reduce the learning curve, making it appealing to newcomers as well as experienced practitioners. A cleaner layout also promotes efficiency in navigating the complex functionalities of MLflow.
- Improved Data Management: Handling datasets can be like juggling hot potatoes; one wrong move, and the whole operation can crumble. Focus on improving data versioning and management will be critical. Users deserve tools that seamlessly integrate data handling with their experiments, reducing friction in the process.
Ending and Key Takeaways
As we wind down our exploration of MLflow and its integration into machine learning workflows, itâs vital to reflect on the journey and highlight the key takeaways from this extensive guide. The significance of approaching MLflow integration with a structured plan is paramount. This section not only encapsulates the insights acquired but also serves as a roadmap for those looking to implement what theyâve learned. Each element discussed plays a crucial role in shaping a well-oiled machine learning project.
Summarizing the Journey
The journey through MLflow has unveiled a rich tapestry of functionality that, when harnessed effectively, can enhance any machine learning project. From understanding the core components such as Tracking, Projects, Models, and Registry to setting up comprehensive workflows that integrate seamlessly, every step is essential. This guide has emphasized the importance of version control and reproducibilityâtwo pillars that stand firm in the complex world of machine learning. Each case study illustrated potential pitfalls and triumphs, showcasing how businesses of all sizes can ride the MLflow wave. With the landscape of machine learning constantly evolving, one might feel overwhelmed; however, remember that each challenge is merely a stepping stone to greater efficiency and discovery.
"Effective MLflow integration isn't just beneficial; it's essential for those seriously venturing into machine learning."
Next Steps for Implementation
Now that youâve wrapped your head around the intricacies of MLflow, turning knowledge into action is the next crucial phase. Start by assessing your current machine learning workflows; identify where MLflow can alleviate inefficiencies. This might involve a few tweaks here and there to your data pipelines or experiment tracking.
- Begin with Small Steps: Donât dive in headfirst. Pilot a small project using MLflow. Get comfortable with the tools before scaling up.
- Collaborate with Your Team: Share knowledge and best practices with your colleagues. Effective collaboration can foster a communal understanding of MLflowâs advantages, making implementation smoother.
- Seek Out Resources: Leverage online communities and resources. Websites like Wikipedia and Reddit can offer valuable insights and real-world applications to widen your perspective.
- Iterate and Improve: Implementation is not a one-time effort. Keep an eye on the outcomes and continuously optimize your use of MLflow for maximum efficiency.
By taking these necessary steps, it ensures a robust and effective integration that not only meets the demands of current projects but also caters to future endeavors in the realm of machine learning. In this evolving space, continual adaptation and learning are what keep you ahead of the curve.