Harnessing RKT Modeling for Effective Decision-Making
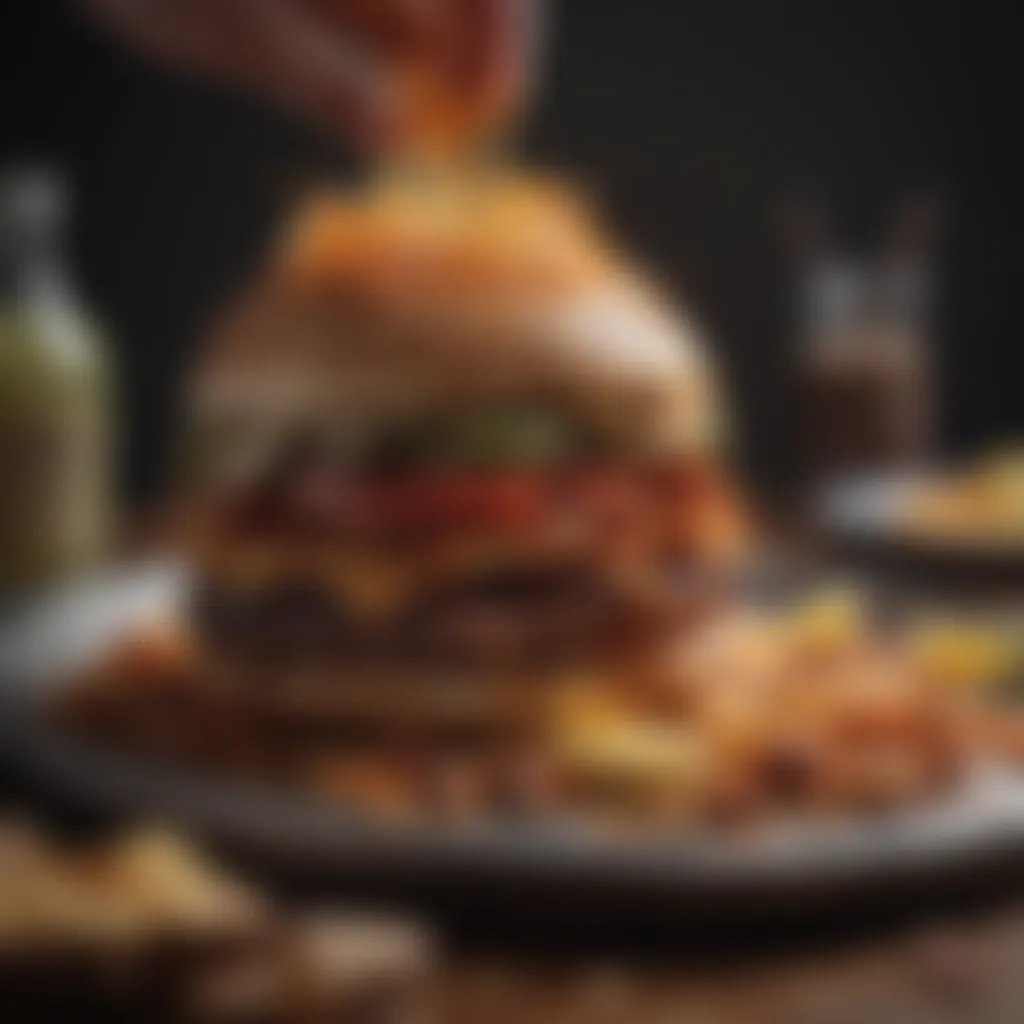
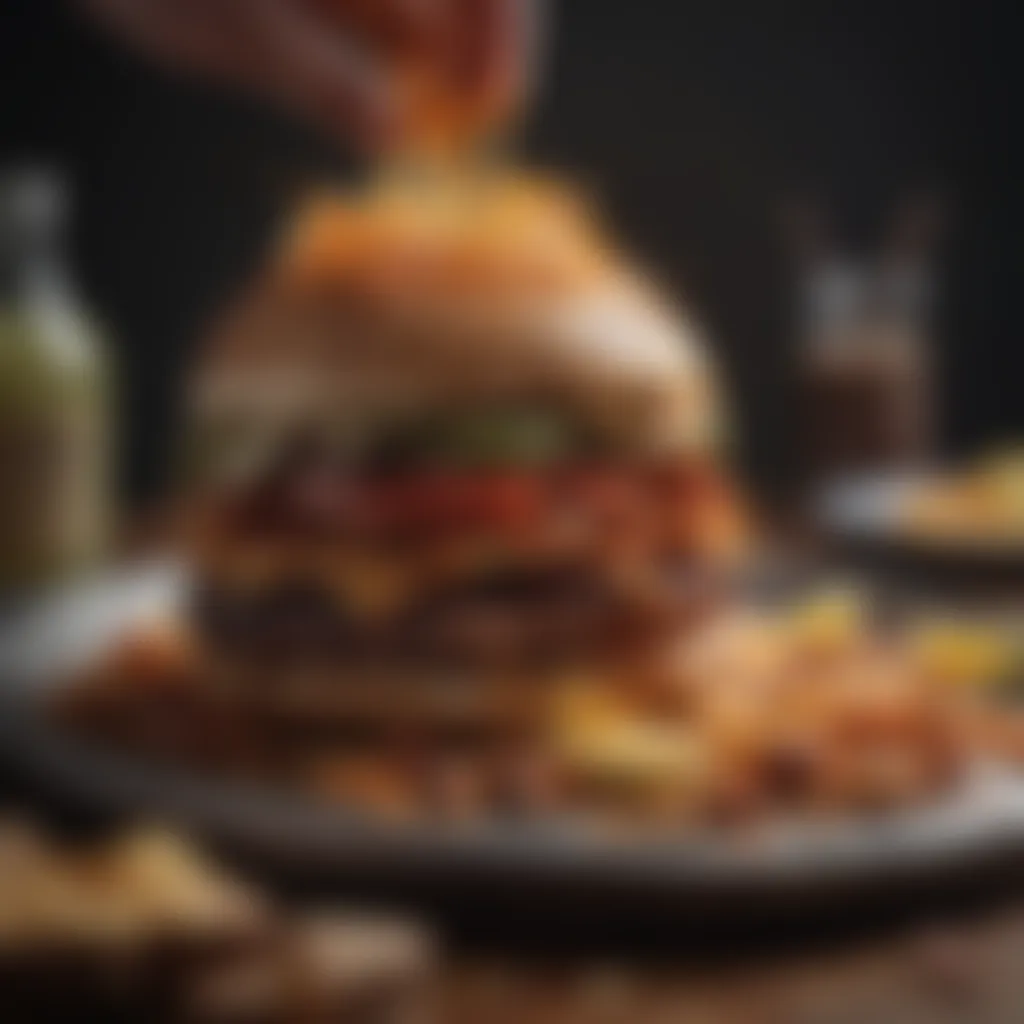
Intro
RKT modeling, an acronym that resonates in the corridors of data analysis and decision-making, is more than just a fancy term among scholars. This approach merges multiple methodologies into a cohesive tool that allows for enhanced insights and more effective strategies across various fields. Gaining a clear picture of RKT requires delving into its core methodologies, benefits, and potential pitfalls.
As we stroll through this comprehensive guide, we aim to unravel the complexities and empower readers—particularly those who navigate their daily worlds seeking to make informed decisions, whether at home, in business, or within scientific arenas. Understanding RKT modeling is like adding a powerful ingredient to your cooking arsenal; once you grasp it, your ability to whip up analytical masterpieces is boundless.
The journey consists of several sections, starting from an overview of the essential components to an exploration of real-world applications and future directions. Each part contributes to a well-rounded understanding, layering knowledge akin to the careful construction of a perfect meal.
Prelude to RKT Modeling
RKT modeling stands as a pivotal concept in decision-making frameworks, particularly when it comes to navigating complex systems. Its ability to weave together various techniques makes it not only powerful but essential for those seeking to enhance their understanding of intricate relationships within data. By integrating mathematical models, statistical methods, and computational power, RKT modeling provides a platform that supports informed choices across different fields, from finance to healthcare.
The primary strength of RKT modeling lies in its flexibility. This adaptability allows practitioners to customize their approach based on the nuances of the data they encounter. Whether you're parsing through financial records or delving into operational research outputs, RKT's structured methodology carries a weight of credibility and insights.
Moreover, embracing RKT modeling opens up avenues for improved risk assessment and forecasting. This is especially valuable in today's fast-paced environment, where data-driven decisions can set organizations apart from their competitors. By grounding conclusions in solid models, users gain confidence in their strategies, paving the way for success with less guesswork.
The importance of understanding RKT modeling cannot be overstated. As complexities grow within various domains, neglecting this framework might lead to missed opportunities and vulnerabilities. Therefore, it's crucial to familiarize oneself with the foundational elements that drive RKT modeling.
Defining RKT Modeling
At its core, RKT modeling stands for Risk, Knowledge, and Theory modeling. It aims to offer a structured means to assess uncertainties and to make informed decisions based on available evidence. In simpler terms, RKT modeling is akin to mapping a road before embarking on a journey—knowing potential pitfalls and alternative routes can significantly alter your travel plan.
RKT modeling integrates various elements:
- Risk Assessment: Identifying uncertainties that may impact outcomes.
- Knowledge Integration: Utilizing existing knowledge bases and frameworks to bolster understanding.
- Theoretical Foundations: Basis of models rooted in established theories for reliability.
This multi-faceted approach allows users to dissect a problem thoroughly, offering insights that are not only timely but also relevant.
Historical Context and Development
A deep dive into the history of RKT modeling reveals a rich tapestry of evolution. To pinpoint its origins, one must travel back several decades when disciplines like statistics and operational research began intertwining with practical applications. The quest to understand uncertainties, particularly in engineering and economic sectors, laid the groundwork for what would eventually become RKT modeling.
In the past, isolated models struggled to convey the complexities of real-world scenarios effectively. This often left decision-makers with fragmented information, hampering their ability to act decisively. However, as academia and industry merged, a need arose for a cohesive framework—thus, RKT modeling began to crystallize.
With contributions from various fields, including behavioral economics and systems theory, RKT evolved into a robust methodology capable of tackling multifaceted challenges. This cross-pollination of ideas fostered a deeper understanding of how to model risks and uncertainties, leading to enhanced analytical tools that can be applied across numerous sectors.
Ultimately, RKT modeling has grown more relevant as the world has become increasingly data-driven. Evaluating its historical roots not only sheds light on its current applications but also highlights the importance of continual adaptation to emerging challenges and technologies that impact decision-making today.
Core Components of RKT Modeling
Understanding the core components of RKT modeling is essential. These elements form the backbone of any successful modeling effort. They help in enhancing clarity, improving decision-making, and fostering a deeper analysis of complex systems. When executed effectively, each element provides a structured approach that allows modelers to address specific problems or questions. This section dives into the vital components that make RKT modeling effective.
Key Variables and Parameters
In RKT modeling, identifying the key variables and parameters is the first step. These elements are the fundamental building blocks around which the entire model is constructed. A variable can be anything from temperature to economic indicators, while parameters often act as constants that help define the relationship between various elements in the model.
By carefully selecting which variables to include, one can significantly impact the effectiveness of the model. High-impact variables typically have strong relationships with the outcome being studied. This might mean looking at historical data or consulting subject matter experts to determine which aspects are crucial. It’s also important to continually reassess these variables as new data comes in, as relationships may change over time.
Data Integration Techniques
Data integration is another crucial component. In a world buzzing with information, having a streamlined way to consolidate different data sources is paramount. It ensures that modelers are not working in silos, thus facilitating a more comprehensive analysis.
There are several techniques for data integration. Traditional methods may involve manually cleaning data, which can be tedious but ensures accuracy. On the other hand, automated tools can facilitate seamless integration by pulling data from various databases or APIs. The choice of technique depends heavily on the specific requirements and constraints of the model in question. Effective data integration allows for enriched insights, which are gold when it comes to making informed decisions.
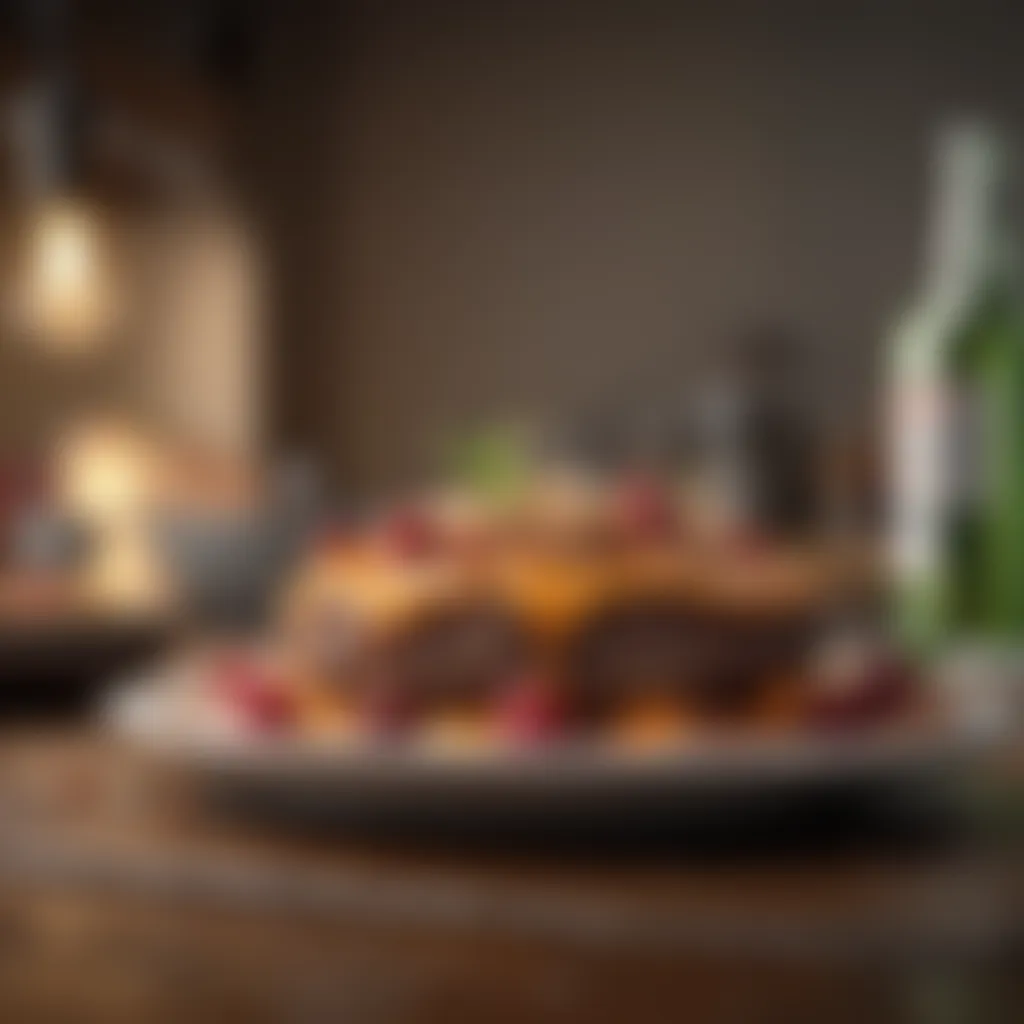
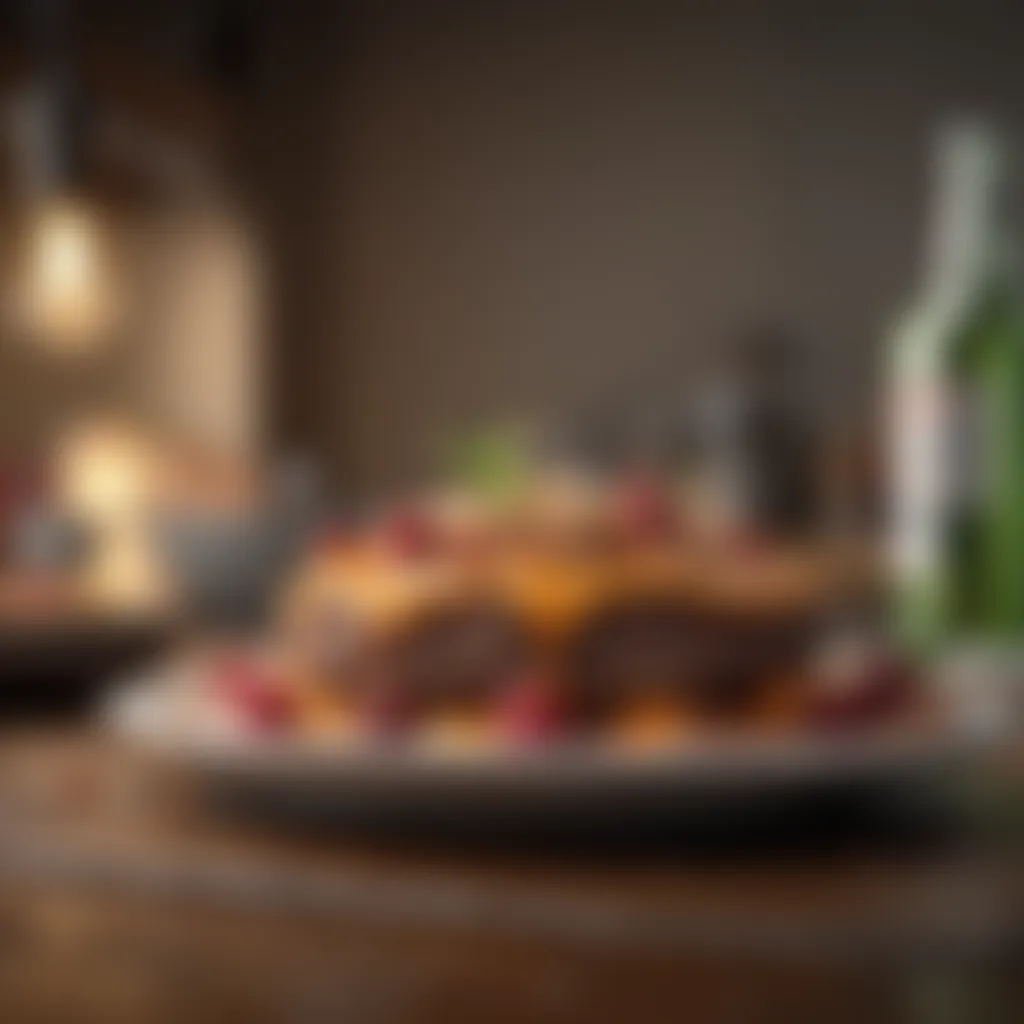
Modeling Algorithms
Diving deeper into RKT modeling, we encounter the various algorithms that enable the processing and analysis of data. These algorithms play a crucial role in determining how the model behaves and what outputs are generated. Let’s discuss three prominent categories of modeling algorithms:
Linear and Non-linear Models
When looking at linear and non-linear models, one can see a clear differentiation in complexity. Linear models, as the name implies, establish a straight-line relationship between variables. They are prized for their simplicity and interpretative ease. Most casual users appreciate how quickly they can work with linear models, making them a popular choice for foundational insights.
Non-linear models, however, capture the complexities of real-world scenarios where relationships are not always straight lines. They can be a bit trickier to handle but allow for an enhanced understanding of intricate datasets. The unique feature of non-linear models is their flexibility to adapt to various datasets, often revealing insights that linear models might overlook. Despite their advantages, non-linear models can become computationally intensive, which should be taken into account.
Probabilistic Approaches
In the realm of probabilistic approaches, one can finally embrace uncertainty. This method allows for exploring various possibilities, providing a broader view of potential outcomes. With a key characteristic being the formulation of probability distributions, this approach effectively helps in estimating risk and variability. It’s a beneficial choice, especially in fields like finance and healthcare where uncertainty is a constant.
The unique feature of probabilistic models is their ability to account for a variety of potential scenarios, rather than sticking to a single deterministic outcome. This can be incredibly valuable but also complicates interpretation. Having to communicate probabilities to stakeholders requires a clear strategy and awareness of how these insights translate into decision-making.
Simulation Methods
Lastly, simulation methods form an integral part of the modeling landscape. These techniques allow for running various scenarios without real-world consequences. A key characteristic of simulation methods is their iterative nature; you can tweak factors and observe different outcomes, which is invaluable in risk assessment.
Simulation comes with its challenges as well. It requires substantial computational power and can be time-consuming. However, the ability to visualize outcomes over a range of variables enhances understanding and aids in making better decisions.
Essentially, the core components of RKT modeling provide the necessary structure to tackle complex questions. Paying attention to these elements can lead to improved models that are both insightful and actionable.
Applications of RKT Modeling
In the world of complex decision-making, the applications of RKT modeling hold considerable significance. This modeling framework provides tools that enhance understanding and analysis across various domains. It isn’t merely a method; it stands as a bridge connecting data with practical insights, guiding informed choices. RKT modeling finds merit in multiple sectors, illuminating pathways where traditional techniques might flounder because of their limitations. These applications underscore the versatility and robustness of RKT modeling.
RKT in Finance
In finance, RKT modeling carves out a niche that is pivotal for effective risk assessment and investment strategies. For instance, when evaluating complex financial instruments like derivatives, RKT helps analysts in identifying correlations and potential outcomes, far beyond the simplistic models of yesteryear. Investors leverage this modeling to sift through vast data sets, uncovering inefficiencies or opportunities that are often hidden from plain sight.
Moreover, financial organizations apply RKT to enhance portfolio management. Through simulation methods, they can predict potential shifts in the market, thus empowering decision-makers to react proactively. Whether it’s predicting market trends or assessing credit risks, RKT serves as a highly adaptable tool, streamlining the decision-making process while ensuring a data-driven approach.
RKT for Risk Management
Risk management is another arena where RKT modeling shines. Companies today face an overwhelming array of risks—financial, operational, strategic, even reputational. Here, RKT plays an integral role in illuminating risk factors that necessitate attention. Using data integration techniques, organizations can compile and analyze data from different sources, allowing for more coherent risk assessments.
For example, in an insurance company, RKT modeling helps in predicting loss ratios and potential claims based on historical data. By utilizing linear and non-linear models, insurers can create robust frameworks that warn against unforeseen losses, aiding in more effective underwriting practices. It’s a blend where data intricacies meet real-world implications, ensuring businesses can navigate the turbulent waters of uncertainty with greater confidence.
Utilization in Operations Research
In operations research, RKT modeling emerges as a valuable approach for optimizing processes. By employing RKT, businesses can identify bottlenecks within their operations, ultimately leading to smoother workflows. This modeling form combines mathematical optimization with real-time analytics to devise strategies that enhance efficiency.
Think about a manufacturing plant trying to streamline production. RKT modeling can analyze each stage of the production line, offering insights on where time and resources are wasted. Using simulation methods, organizations can create different scenarios to figure out the best strategy for resource allocation. Hence, RKT becomes not just a modeling approach but a strategic partner in refining operational systems.
Healthcare Applications
In healthcare, the relevance of RKT modeling cannot be overstated. It serves as a critical tool in predicting patient outcomes and optimizing treatment plans. For instance, hospitals utilize this model to analyze patient data, which culminates in better resource management and improved patient care.
With the integration of big data and machine learning techniques, healthcare providers can proactively address potential issues. If a hospital notices an uptick in a specific health condition among its patients, RKT can help analyze underlying causes, leading to timely interventions. The collaborative angle of RKT modeling ensures that the healthcare sector is not just reactive but also proactive in safeguarding public health.
Impact on Environmental Studies
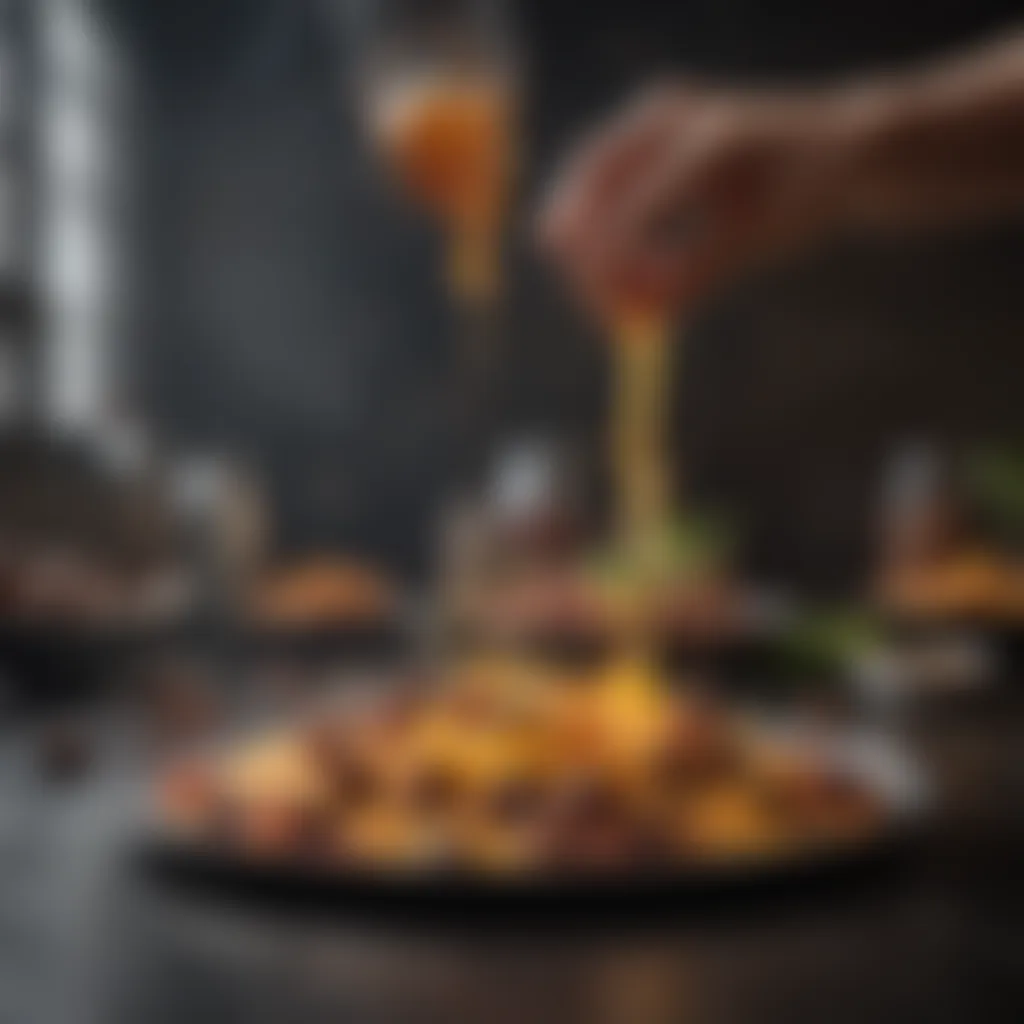
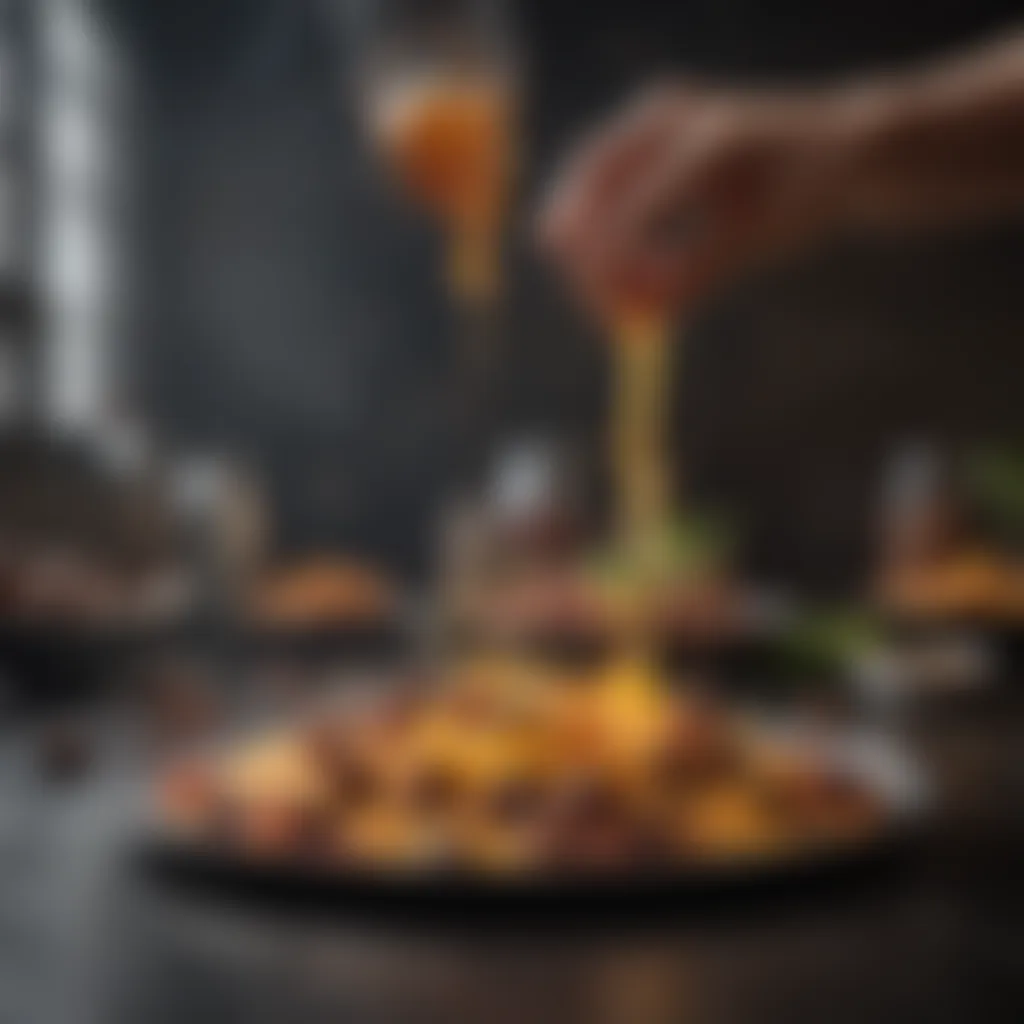
RKT modeling also extends its usefulness to environmental studies. With pressing challenges like climate change, pollution, and resource depletion, RKT becomes invaluable for modeling ecological impacts and forecasting environmental changes. Researchers employ RKT techniques to analyze various environmental factors, developing predictive models to understand potential outcomes.
For example, RKT can simulate different scenarios based on varying levels of pollution emissions, enabling scientists to project future environmental conditions. These insights arm policymakers with the knowledge needed to craft regulations that protect the environment. By blending scientific understanding with robust data analysis, RKT modeling facilitates informed decision-making that aligns both human needs and environmental sustainability.
Methodological Challenges in RKT Modeling
RKT modeling, while versatile and impactful, does not glide through all processes without its set of challenges. These hurdles can significantly affect the quality and applicability of results derived from such models. Understanding the various methodological challenges associated with RKT modeling is crucial for anyone looking to implement this framework effectively. By tackling these challenges head-on, we not only improve our models but also make them more aligned with real-world dynamics. This section will explore three primary challenges: data quality and accessibility, model complexity and interpretability, and computational constraints.
Data Quality and Accessibility
Good data is the foundation of any successful model. In RKT modeling, poor quality data can lead to skewed results, which may misinform decision-making. Data comes from various sources, and ensuring its accuracy is no small feat.
- Inconsistencies in datasets can arise from different data collection methods. For instance, consider a model predicting household spending based on surveys. Some respondents might report spending differently depending on their understanding or interpretation of the questions asked. These discrepancies can cause confusion in the data pool.
- Accessibility is another concern. Some valuable datasets are locked behind paywalls or require special permissions. This lack of transparency can hinder researchers or practitioners who are eager to apply RKT modeling in their respective fields. Additionally, even within the same organization, teams might not share data uniformly, leading to the infamous "silo" mode where information remains trapped, limiting the benefits of integrated analyses.
In summary, addressing data quality and ensuring accessibility are paramount for establishing the credibility and reliability of RKT modeling efforts. \n
"Data is the new oil, but like oil, we need the right tools to extract and refine it."
Model Complexity and Interpretability
With great power comes great responsibility—this couldn't be truer in the case of RKT modeling. As models become more sophisticated, their complexity can create barriers to interpretation. Stakeholders need to understand not just the outcomes but also the 'why' and 'how' behind those results.
- High complexity models often involve numerous variables and intricate relationships, making them difficult to interpret. For example, a multivariate model predicting educational outcomes could rely on a plethora of factors ranging from socioeconomic status to teaching methods. For policymakers, if they do not grasp how each factor interacts, they may miss key insights.
- Interpretable models are crucial, especially in sensitive fields like healthcare or finance, where decisions can have lasting repercussions. If a model suggests a specific treatment plan without clear reasoning, healthcare professionals might hesitate, leading to potential delays or even errors in patient care.
Thus, finding the balance between advanced modeling and interpretability is a challenging yet necessary endeavor for those employing RKT methodologies.
Computational Constraints
In a world increasingly reliant on data and analytics, computational power is crucial. However, RKT modeling can stretch the limits of available computational resources.
- Scalability often presents a barrier. As datasets grow larger, the algorithms handling them may falter or slow down significantly. Picture this scenario: an online retailer trying to customize offers based on user behavior. If the underlying model takes too long to compute recommendations, businesses risk losing potential sales while waiting for insights to materialize.
- Resource efficiency must also be considered. Not every organization has access to advanced supercomputers or cloud services. For smaller entities, the computational demands of certain RKT models might be daunting and unaffordable, limiting their ability to compete in data-driven decision-making landscape.
As we work through the obstacles posed by computational constraints, finding innovative ways to optimize algorithms will be fundamental in making RKT modeling more accessible and efficient for broader audiences.
Future Directions for RKT Modeling
The landscape of RKT modeling is constantly evolving, and understanding its future directions is pivotal for professionals and enthusiasts alike. This section hones in on the emerging trends shaping the field, highlighting vital advancements, potential integrations, and immediate developments that are essential for effective decision-making in varied environments. This forward-looking exploration underscores how upcoming changes will not only enhance modeling accuracy but also broaden its applicability across domains.
Advancements in Machine Learning Techniques
Machine learning has become a game changer in RKT modeling. With the capacity to analyze large datasets and discern patterns that elude traditional methods, these techniques are transforming the way practitioners approach model development.
Some promising developments include:
- Deep Learning: This method leverages neural networks to improve predictive capabilities. By mimicking the human brain’s processing, deep learning algorithms can better handle complex data inputs.
- Automated Modeling: Tools now exist that automate the model selection and tuning process. This not only saves time but significantly reduces the potential for human error.
The integration of machine learning fosters greater model resilience, efficiency, and ultimately, accuracy.
Integration with Big Data
In our era of information overload, the interplay between RKT modeling and big data cannot be overstated. The sheer volume of data being generated presents a rich tapestry for modelers, but it also brings challenges regarding interpretation and relevance.
Utilizing big data involves:
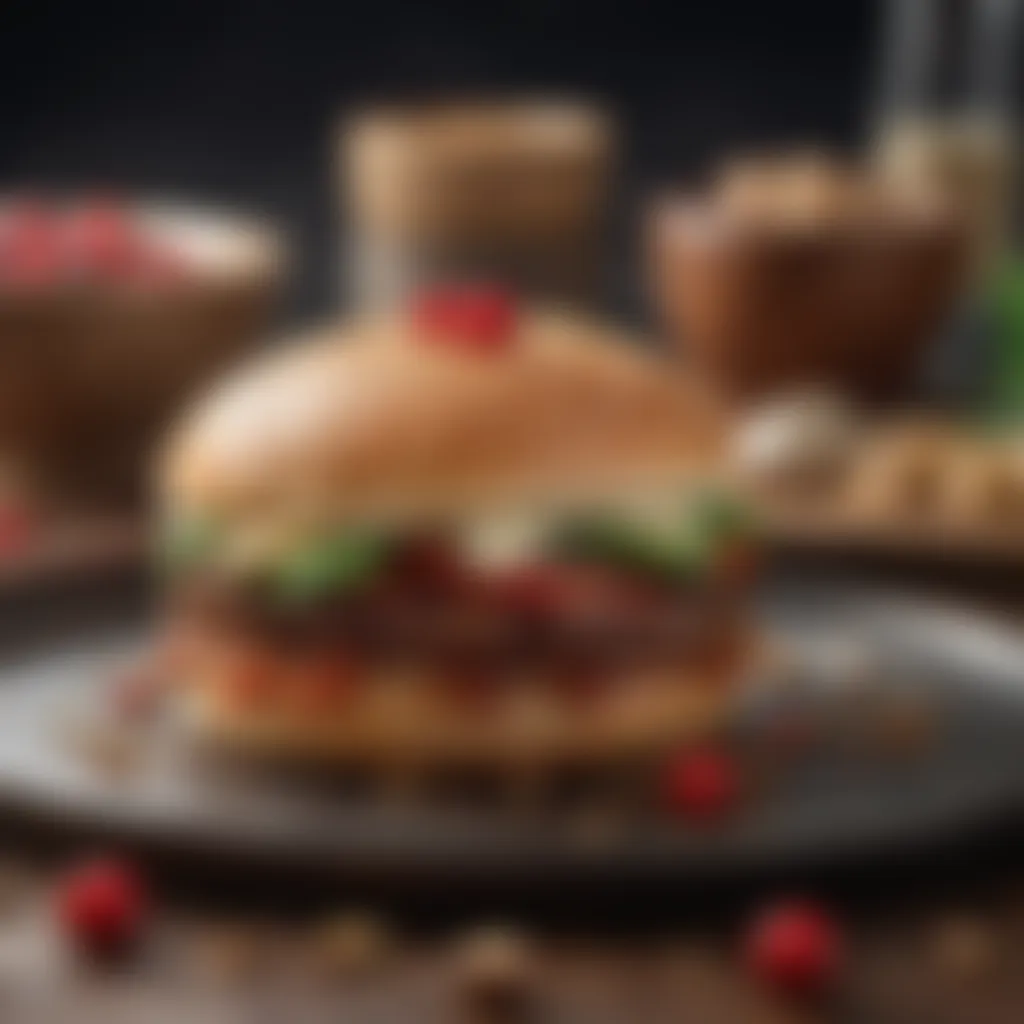
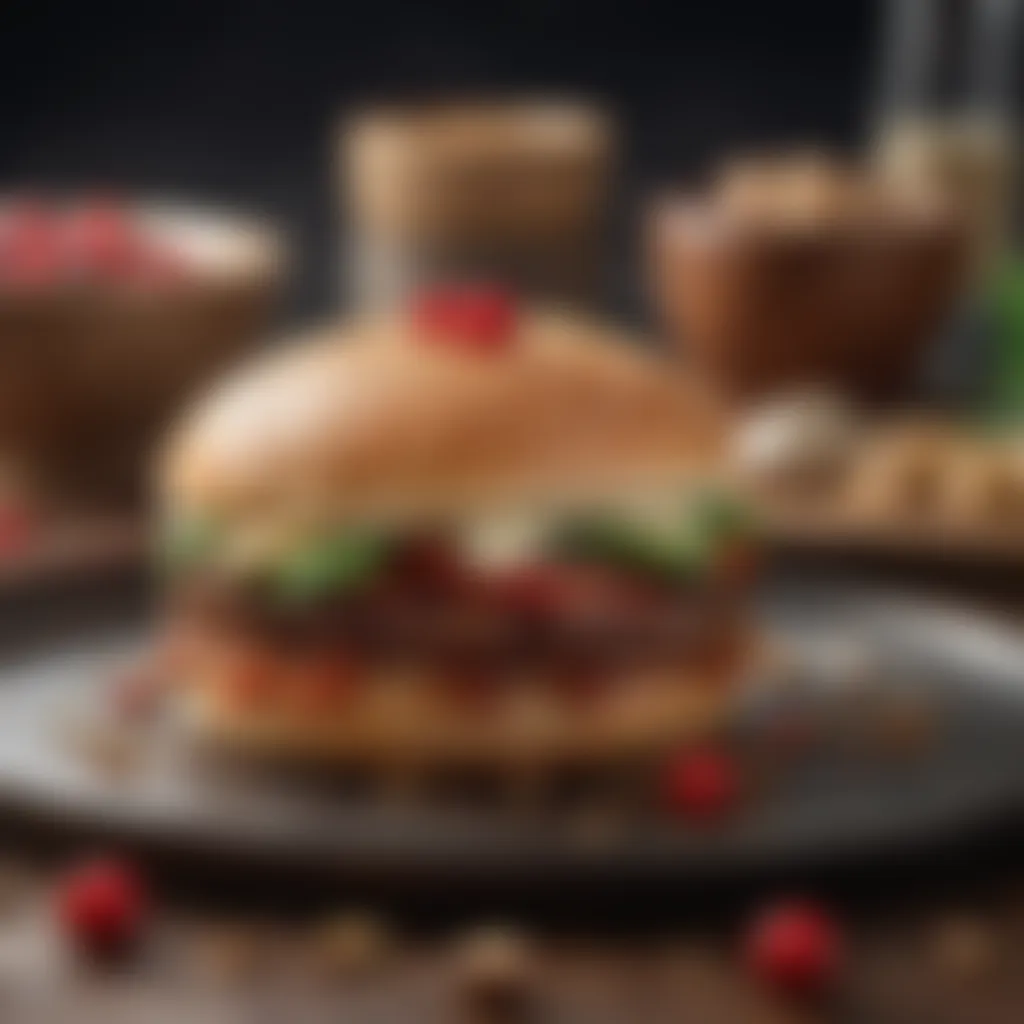
- Enhanced Predictive Power: With access to larger datasets, models have the potential to improve their reliability and precision. This is particularly beneficial in fields like finance and healthcare where stakes are high.
- Tailored Approaches: The granularity of big data allows for more personalized modeling frameworks, catering to niche markets or specific user needs.
As systems become integrated with big data, the flexibility and scope of RKT modeling will grow remarkably.
Real-time Data Processing
To stay ahead, RKT modeling must adapt to the increasing demand for real-time insights. This shift towards immediacy can dramatically alter how decisions are made in fast-paced environments.
Real-time processing entails:
- Instantaneous Insights: The capability to analyze data as it flows can lead to quicker, more informed decision-making. Businesses can swiftly react to market changes, while healthcare practitioners can respond quickly to patient needs.
- Continuous Learning: Models can continually adapt based on incoming data, allowing for strategies that evolve rather than remain static over time.
These advancements will not only improve how RKT models serve their users but also enhance their relevance in today's rapidly-changing context.
Key Point: The future of RKT modeling hinges on its ability to embrace machine learning, leverage big data, and cultivate real-time analysis. These elements will be essential in shaping the next generation of decision-making tools.
Ethics and Responsible Use of RKT Modeling
Understanding the ethical frameworks surrounding RKT modeling is paramount in today’s data-centric world. The responsible use of such powerful modeling techniques ensures that the insights we gain do not come at the expense of privacy, fairness, or societal trust. This section will explore the crucial aspects of ethics in RKT modeling, diving into the specific elements of data privacy concerns and the potential for bias in modeling outputs.
Data Privacy Concerns
In an age where information is akin to currency, data privacy takes center stage in discussions around RKT modeling. User data, whether it's about buying habits or health records, must be treated like a delicate flower—prized and protected. The moment personal data is mishandled, it becomes a leaky ship, risking not only individual privacy but also the credibility of the organizations that utilize RKT models.
While RKT modeling can deliver significant insights for decision-making, it walks a tightrope. A misstep regarding data acquisition or consent can spiral into a jumble of legal consequences and ruined reputations. Thus, organizations should ensure adherence to regulations like GDPR (General Data Protection Regulation) and CCPA (California Consumer Privacy Act). Fires often start from small sparks: neglecting to anonymize user data or skipping over explicit consent can lead to potential breaches. A few key considerations include:
- Informed Consent: Always inform data subjects about how their data will be used.
- Anonymization: Obscuring personal identifiers in datasets helps maintain privacy.
- Data Minimization: Only collect data that is essential for your modeling.
"A strong data privacy strategy helps maintain user trust, which is often as valuable as the data itself."
Bias in Modeling Outputs
Bias in modeling outputs represents another ethical quagmire. RKT models reflect their inputs; thus, if the data fed into the model is skewed or unrepresentative, the results inevitably follow suit. This can lead to incorrect conclusions, which can perpetuate stereotypes or unfairly disadvantage particular groups. The stakes are even higher in sensitive applications like healthcare or criminal justice systems, where biased outputs can significantly impact lives.
Some common sources of bias include:
- Sampling Bias: If the sample isn’t diverse, the model will render narrow views.
- Confirmation Bias: Developers might unconsciously lean toward data that supports their hypotheses, overlooking contradictory evidence.
- Historical Bias: Using outdated data can reinforce existing disparities in the models made.
To counteract bias, transparency must be the name of the game. Involve diverse teams in data collection and modeling to shine light on hidden biases. Moreover, rigorous testing can help identify and mitigate potential biases before the model reaches decision-makers.
In closing, the ethical implications of RKT modeling underline a profound responsibility that organizations must shoulder. From ensuring data privacy to actively fighting biases, holding the ethical torch high ensures that the benefits of RKT modeling are realized without sacrificing fairness or trust.
End
As we reach the end of our comprehensive exploration of RKT modeling, it's essential to recognize the significance of this thematic wrap-up. The conclusion serves not just to echo previously discussed points but also to crystallize the knowledge gained throughout the article. This is where we make sense of the various threads we’ve woven together, examining the multifaceted implications it has on decision-making and analysis.
Recap of Key Insights
First and foremost, RKT modeling stands as a powerful framework that harmonizes various methodologies to aid in better decision-making. By dissecting components like key variables and parameters, we have seen how flexibility and precision can coexist in a model. Moreover, the applications of RKT modeling across diverse fields—from finance to healthcare and environmental studies—illustrate its versatility and relevance in solving real-world problems.
We also delved into methodological challenges such as data quality, model complexity, and computational limits. Recognizing these hurdles can guide future research and application, ensuring that RKT remains a robust tool well into the future. Identifying data privacy and biases has highlighted the ethical dimensions we must navigate as practitioners in this field. Insightfully, these components can dramatically influence the outcomes derived from these modeling approaches.
Final Thoughts on RKT Modeling
Looking ahead, RKT modeling is poised for significant growth, especially with the integration of machine learning and big data technologies. As we continue to refine our approaches, the possibilities for real-time data processing present exciting opportunities. However, this potential brings with it responsibility: ensuring ethical practices, maintaining data integrity, and filtering out biases in our models will be paramount to advancing the field responsibly.
The future of RKT modeling is not just about enhancing analytical capabilities, but also ensuring that our methodologies reflect a commitment to ethical standards and inclusivity in data representation.
In summary, as we close this chapter on RKT modeling, remember that the landscape is constantly evolving. Staying abreast of advancements and ethical considerations will empower you to leverage the benefits of RKT modeling effectively, crafting better strategies for whatever domain you choose to apply it. The insights shared here are not just for understanding RKT modeling but are stepping stones for your journey towards mastering its application.